Aerial Object Detection: Deep learning approaches and applications
- Chapter- 1 Introduction
- Chapter- 2 Oriented Object Detection
- Chapter- 3 DOTA: One of the largest datasets in aerial object detection
- Chapter- 4 Breakthrough techniques in Aerial Object Detection
- Chapter- 5 Training state-of-the-art models with the DOTA dataset
- Chapter- 6 Model architectures
- Chapter- 7 Model performances
- Chapter- 8 Result
- Chapter- 9 Applications
1. Introduction
Aerial object detection is a breakthrough technique in deep learning and has gained remarkable significance in various industries, revolutionizing the way specific objects are tracked and analyzed in images or video streams. Object detection in natural images has witnessed significant advancements however the complexities of aerial images present unique challenges that require tailored approaches. While natural images capture objects from various profiles such as front view or side view, aerial images, usually taken by satellites or drones, provide a top-down view that emphasizes the roof information of geospatial objects
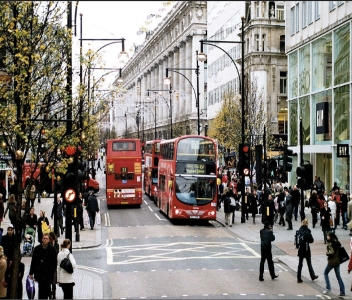
Fig 1: Natural Image1
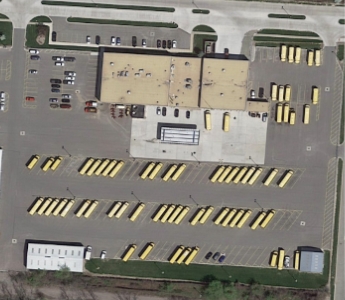
Fig 2: Aerial Image1
The distinctive characteristics of aerial images such as arbitrary orientations, scale variations, nonuniform object densities, and large aspect ratios make image interpretation a challenging task. At present, various Earth Vision technologies enable us to observe Earth’s surface with aerial images. Specific mathematical tools and numerical algorithms are necessary for interpreting these images since they are typically very huge in resolution. The right model for aerial object detection will help us facilitate the critical role it plays in real-world applications such as urban planning, agriculture management, disaster relief, and surveillance
2. Oriented Object Detection
Oriented object detection involves detecting and localizing objects in images or videos while also estimating their orientation or rotation. The arbitrary orientation of objects caused by overhead view is the main difference between natural images and aerial images. It causes two major problems.
- Pre-trained object detection models trained on natural images fail badly on aerial images
- Horizontal Bounding Box (HBB) representation used in conventional object detection cannot locate oriented objects precisely
Oriented Bounding Box (OBB) representation is more accurate for aerial images
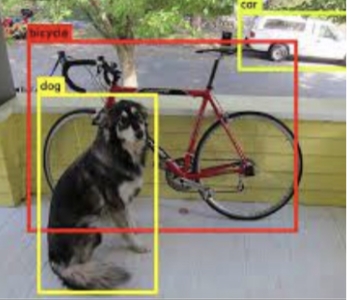
Fig 3: Horizontal Bounding Box1
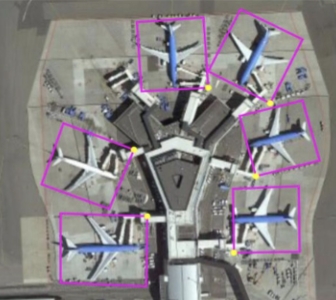
Fig 4: Oriented Bounding Box1
Another issue with object detection on aerial images is that the module design and the hyperparameter setting of conventional object detectors learned from natural images are not appropriate for aerial images due to domain differences. Current object detection libraries such as MMDetection and Detectron do not support oriented object detection.
The OBB object representation introduces a new object detection task, called oriented object detection. Unlike horizontal object detection, this approach enables the detection of objects with specific orientations. Most of the approaches developed for this task aim to leverage the success of deep learning-based object detectors that have been pre-trained on extensive datasets of natural images.
To train a deep aerial image detection model we must need a dataset with oriented bounding box annotation. For a horizontal bounding box, a common representation of the bounding box is (xc, yc, w, h), where (xc, yc) is the center location and w, h are the width and height, respectively.
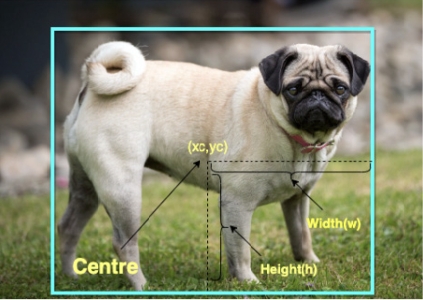
Fig 5: HBB representation1
A horizontal bounding box can describe objects well in most cases. However, it cannot accurately outline oriented instances in aerial images as shown in this figure
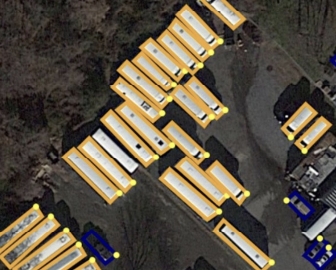
Fig 6: HBB Annotation in aerial image2
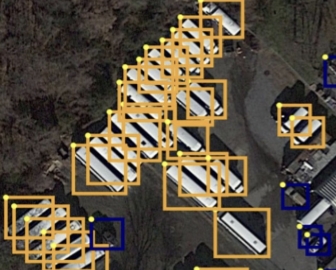
Fig 7: OBB Annotation in aerial image2
To annotate oriented objects better, OBBs are denoted as (x1,y1,x2,y2,x3,y3,x4,y4) where (x1,y1), (x2,y2), (x3,y3) and (x4,y4) are four corner points of the bounding box arranged clockwise. This object can also be represented as (x2,y2,x3,y3,x4,y4,x1,y1) where the same arrangement is maintained. To avoid this confusion we take the corner with the highest vertical coordinate (head of the object) as the starting point
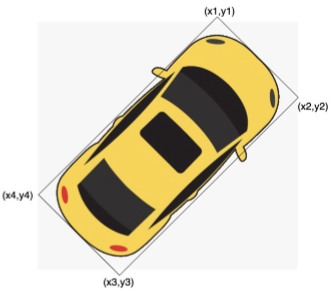
Fig 8: OBB denotation1
3. DOTA: One of the largest datasets in aerial object detection
In aerial object detection, there are only a few annotated datasets available. However, these datasets often have a limited number of instances and predominantly use images captured under ideal conditions. The most extensive dataset for object detection in Earth Vision is the expanded DOTA dataset. It not only contains a large number of instances but also includes annotations for oriented bounding boxes. Let’s delve into the DOTA dataset to understand it better.
The DOTA dataset gathers images from various sources, including Google Earth, GF-2 and JL-1 satellites operated by the China Center for Resources Satellite Data and Application, and aerial images provided by CycloMedia B.V. All these images consist of both RGB (colored) and grayscale formats. However, RGB images are sourced from Google Earth and CycloMedia, while the grayscale images are derived from the panchromatic band of GF-2 and JL-1 satellite images. All images are stored in the ‘png’ format for convenient access and analysis.

Overview
The DOTA dataset contains 15 common categories, 2806 images, and 188282 instances. Classes are namely - plane, ship, storage tank, baseball diamond, tennis court, basketball court, ground track field, harbor, bridge, large vehicle, small vehicle, helicopter, roundabout, soccer ball field, and swimming pool.

Image size
DOTA images are in the range of 800X800 to 20000X20000 pixels and contain objects exhibiting a wide variety of scales, orientations, and shapes.

Annotation format
- Each object is annotated by an oriented bounding box, which can be denoted as (x1,y1,x2,y2,x3,y3,x4,y4), where (xi,yi) denotes the i-th vertices of OBB. The vertices are arranged in a clockwise order.
- Apart from OBB, each instance is also labeled with a category and a difficulty which indicates whether the instance is difficult to be detected (1 for difficult, 0 for not difficult). Annotations for an image are saved in a text file with the same file name. Each line represents an instance.
x1, y1, x2, y2, x3, y3, x4, y4, category, difficult
x1, y1, x2, y2, x3, y3, x4, y4, category, difficult
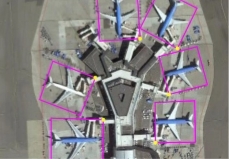
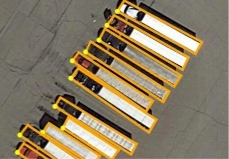
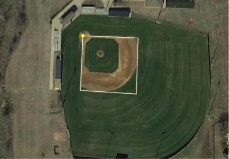
Fig 9: Annotated DOTA images3
4. Breakthrough techniques in Aerial Object Detection
In this section, we will discuss some of the most recent deep learning-based aerial object recognition algorithms since we have a fundamental grasp of aerial object detection problems and training datasets. With the development of deep learning, many researchers in Earth Vision have adapted deep object detectors developed for natural images to aerial images. Here, we highlight some notable advancements.
Rotation-Invariant Layer into Region-Convolutional Neural Network (R-CNN)
Objects in aerial images are often arbitrarily oriented due to the bird’s-eye view, and the scale variations are larger than those in natural images. To handle rotation variations, a simple model plugs an additional rotation-invariant layer into R-CNN relying on rotation data augmentation.
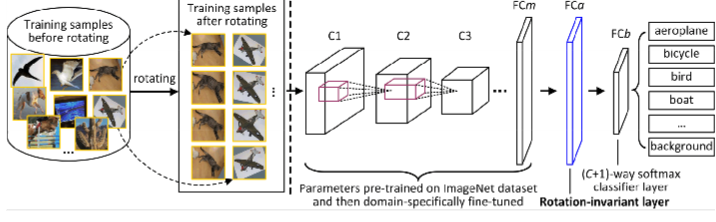
Fig 10: Integrating Rotation-Invariant Layer4
Oriented Response Network
It introduces Active Rotating Filters (ARF) to produce the rotation-invariant feature without using data augmentation, which is adopted by the rotation-sensitive regression detector
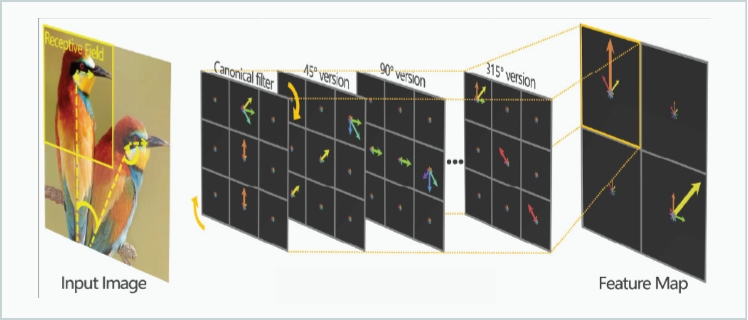
Fig 11: Integrating Active Rotating Filter5
Rotation Region of Interest
When OBB annotations are available, a Rotation R-CNN (RRCNN) uses Rotation Region-of-Interest (RRoI) pooling to extract rotation-invariant region features.
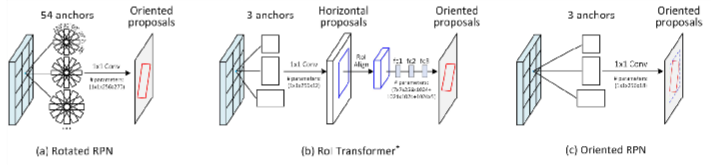
Fig 12: Implementing RRoI6
Feature Pyramids & Image Pyramids
These are widely used to extract scale-invariant features in aerial images
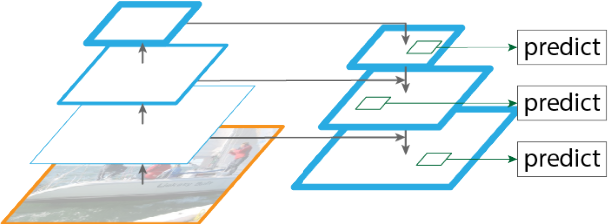
Fig 13: Extracting scale-invariant image features7
Rotated Non-Maximum Suppression (NMS)
Crowded instances represented by HBBs are difficult to distinguish. Traditional HBB-based Non-maximum Suppression (NMS) will fail in such cases. Therefore, some architectures use Rotated NMS (R-NMS), which requires precise detections to address this problem.
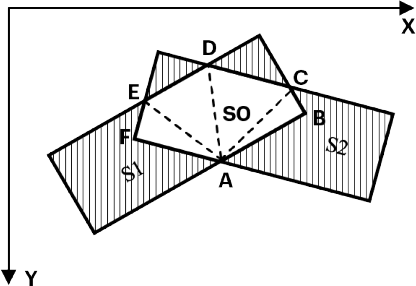
Fig 14: Intersection area between two rotated rectangles8
Mask OBB and CenerMap
Considers object detection as a pixel-level classification problem. Mask-based methods converge more easily but have more Floating Point Operations Per Second (FLOPS) than regression-based methods.
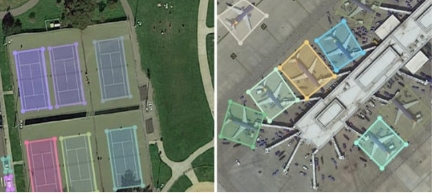
Fig 15: Oriented segmentation9
Split patches
The final challenge is detecting objects in large images. Aerial images are usually extremely large (over 20k × 20k pixels). Current GPU memory capacity is insufficient to process large images and downsampling a large image to a small size would result in the loss of detailed information. To solve this problem the large images can be simply split into small patches. After obtaining the results on these patches, the results are integrated into large images.
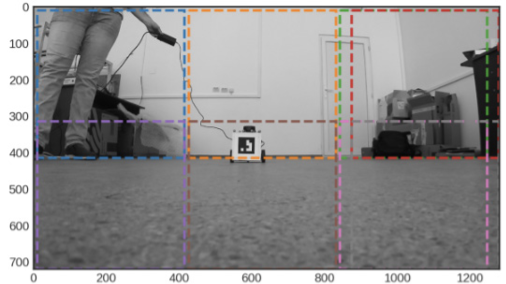
Fig 16: Image split inro smaller parts10
5. Training state-of-the-art models with the DOTA dataset
In our research and survey, we have tested some state-of-the-art deep learning architectures that implement one or more techniques mentioned above to achieve good performance. We have modified the architectures at places to train them on the DOTA dataset. The major objective of this exercise was to discover different cutting-edge techniques that people have worked on for object detection of aerial images and tweak the models wherever needed to generate better results.
We have examined various deep-learning architectures to achieve this goal. To improve the image quality, we have utilized techniques such as denoising, thresholding, histogram equalization, and more. Additionally, we have expanded the training set by applying operations like resizing, flipping, padding, and rotation at random angles.
Model training environment
High computational power was required to examine the deep neural network models due to their complex architecture and the large memory size of the dataset. Hence, we have used AWS EC2 g3.4xlarge/g3.8xlarge/P3.2xlarge engines for model training which come with built-in NVIDIA GPUs. Whenever possible, Spot instances were leveraged to reduce cloud costs to a great extent. As the deep learning framework, PyTorch was used for all our research and exploration.

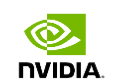
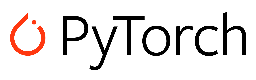
Model development frameworks
Object detection is not a straightforward task, it comes with a good number of complex computations that take place behind the scenes. We already have prebuilt robust frameworks available both from Tensorflow and PyTorch for object detection. For example, Tensorflow Object Detection from Tensorflow and MMdetection & Detectron2 from PyTorch were available. These frameworks are effective in terms of model development and supporting different model architectures. But they lack the modules to support oriented object detection, which is required for aerial object detection, as we have seen in previous sections. For this reason, we have resorted to OBBDetection, which is a customized object detection toolbox derived from MMDetection. Some of the features of OBBDetection are:

Flexible representation of oriented boxes:
This toolbox supports Horizontal bounding boxes, oriented bounding boxes, and 4-point boxes (POLY).

Functionality to train with very high-dimensional images:
This framework has the capability to split huge images into small chunks and join the results together afterward.

Support for multiple out-of-the-box architectures:
This supports various object detection frameworks such as Faster RCNN, RetinaNet, etc along with different backbones.
Pre-processing steps
There are various preprocessing tasks that were executed before doing the actual training.
- Aerial images are usually large in size and for efficient training these need to be split into smaller patches. For our training purposes, we split images into sizes of 1024x1024.
- To increase the overall sample size of the training set and to enhance training accuracy images were scaled to different sizes. These were randomly flipped and rotated. Further normalization operations were also performed and edge images were padded.
6. Model architectures
During our extensive exploration and experimentation, we thoroughly evaluated three different architectures for object detection: Oriented R-CNN, RetinaNet OBB, and YOLO OBB. The primary objective was to identify the architecture that would deliver the highest performance and accuracy in object detection. Out of the three, the Oriented R-CNN architecture-based model exhibited the most exceptional performance.
Oriented R-CNN
We used pre-trained Faster_rcnn_orpn_r50_fpn_1x_ms_rr_dota10 model and retrained with DOTA 2 dataset.
- This architecture resembles Faster RCNN with an oriented region proposal network (ORPN)
- It has ResNet50 as backbone
- Feature Pyramid Network(FPN) is used to generate a pyramid of scaled features
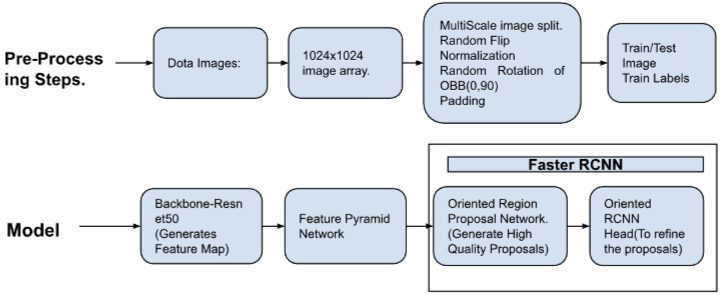
Fig 17: Architecture for Oriented R-CNN11
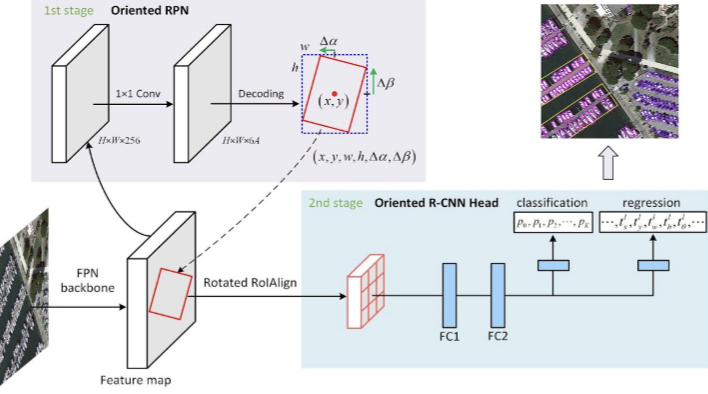
Fig 18: Oriented RCNN as 2 stage detector12
RetinaNet OBB
The highest accuracy object detectors till date are based on a two-stage approach popularized by R-CNN, where a classifier is applied to a sparse set of candidate object locations. In contrast, one-stage detectors that are applied over a regular, dense sampling of possible object locations have the potential to be faster and simpler. RetinaNet OBB is a single stage detector with feature refinement for rotating objects.
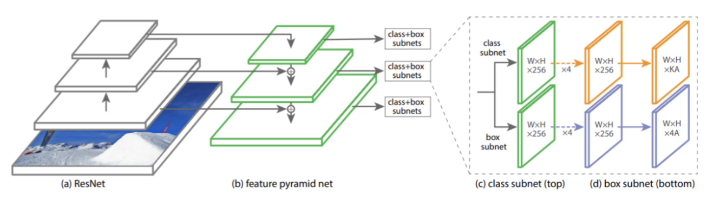
Fig 19: Single stage architecture for RetinaNet OBB13
YOLO OBB
YOLOv5 is one of the most advanced single-stage object detectors. In YOLO OBB, it is extended to oriented object detection.
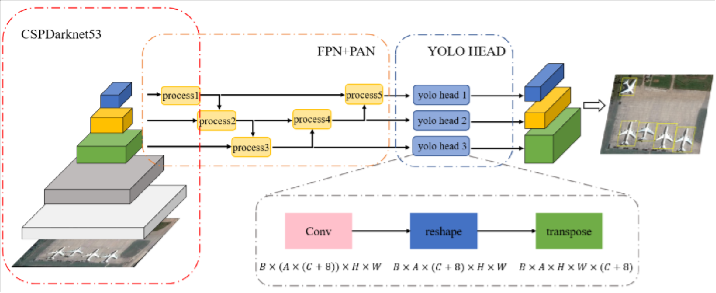
Fig 20: Architecture for YOLO OBB14
Configuration changes
A typical model config file in the OBBDetection framework looks like the one below. We can make changes to the architecture and training setup by changing the parameters in the config file.
We have trained models by setting up different values to multiple parameters. We played around with parameters such as “depth” in “backbone”; “scales”, “ratios”, “strides” in “anchor_generator”; “extend_factor”, “roi_feat_size” in “roi_head” etc.
model = dict( type=’OrientedRCNN’, pretrained=’torchvision://resnet50’, backbone=dict( type=’ResNet’, depth=50, num_stages=4, out_indices=(0, 1, 2, 3), frozen_stages=1, norm_cfg=dict(type=’BN’, requires_grad=True), norm_eval=True, style=’pytorch’), neck=dict( type=’FPN’, in_channels=[256, 512, 1024, 2048], out_channels=256, num_outs=5), rpn_head=dict( type=’OrientedRPNHead’, in_channels=256, feat_channels=256, anchor_generator=dict( type=’AnchorGenerator’, scales=[8], ratios=[0.5, 1.0, 2.0], strides=[4, 8, 16, 32, 64]), bbox_coder=dict( type=’MidpointOffsetCoder’, target_means=[.0, .0, .0, .0, .0, .0], target_stds=[1.0, 1.0, 1.0, 1.0, 0.5, 0.5]), loss_cls=dict( type=’CrossEntropyLoss’, use_sigmoid=True, loss_weight=1.0), loss_bbox=dict(type=’SmoothL1Loss’, beta=1.0 / 9.0, loss_weight=1.0)), roi_head=dict( type=’OBBStandardRoIHead’, bbox_roi_extractor=dict( type=’OBBSingleRoIExtractor’, roi_layer=dict(type=’RoIAlignRotated’, out_size=7, sample_num=2), out_channels=256, extend_factor=(1.4, 1.2), featmap_strides=[4, 8, 16, 32]), bbox_head=dict( type=’OBBShared2FCBBoxHead’, start_bbox_type=’obb’, end_bbox_type=’obb’, in_channels=256, fc_out_channels=1024, roi_feat_size=7, num_classes=15, bbox_coder=dict( type=’OBB2OBBDeltaXYWHTCoder’, target_means=[0., 0., 0., 0., 0.], target_stds=[0.1, 0.1, 0.2, 0.2, 0.1]), reg_class_agnostic=True, loss_cls=dict( type=’CrossEntropyLoss’, use_sigmoid=False, loss_weight=1.0), loss_bbox=dict(type=’SmoothL1Loss’, beta=1.0, loss_weight=1.0))))
7. Model performances
Model performances depend on various factors such as:
- Number of instances in a particular category
- Clarity and visibility of different object categories across images
- Currently available annotations
The total runtime of the model is determined by the available GPUs for training. We utilized AWS instances such as g3.4xlarge, g3.8xlarge, and P3.2xlarge. For our DOTA training, the overall duration ranged from 30 to 35 hours, depending on the specific model used. Our main considerations were efficient speed and cost-effectiveness.
We have trained the models on the DOTA dataset with different modifications. As a performance measure, we have chosen Mean Average Precision (mAP). In this accuracy measure, Average Precision of different classes are calculated for different Intersection over Union (IoU) thresholds and then the mean value of all the average precisions are measured.
The below table shows the performances of the best models.
Oriented RCNN | RetinaNet OBB | YOLO OBB | |
---|---|---|---|
large-vehicle |
0.88 |
0.78 |
0.77 |
swimming pool |
0.79 |
0.73 |
0.74 |
helicopter |
0.92 |
0.76 |
0.72 |
bridge |
0.78 |
0.71 |
0.74 |
plane |
0.9 |
0.72 |
0.71 |
ship |
0.87 |
0.73 |
0.72 |
soccer-ball-field |
0.87 |
0.82 |
0.83 |
basketball-court |
0.94 |
0.81 |
0.82 |
ground-track-field |
0.86 |
0.81 |
0.73 |
small-vehicle |
0.8 |
0.71 |
0.65 |
baseball-diamond |
0.89 |
0.72 |
0.68 |
tennis-court |
0.9 |
0.82 |
0.74 |
roundabout |
0.87 |
0.75 |
0.75 |
storage-tank |
0.9 |
0.82 |
0.83 |
harbor |
0.88 |
0.74 |
0.7 |
mAP |
0.87 |
0.762 |
0.742 |
One can observe there is significant variation across different categories available, there can be multiple reasons for these:
- Categories like basketball court, tennis court, storage tank have very high mAP scores because in a satellite image these take considerable pixel area and are very unique in terms of dimensions and distinctiveness and hence are easy for a neutral network to identify.
- Categories like vehicles are very large in number and looking at area coverage by a single unit is very small. This leads to the ambiguity of a neural network to identify various small objects present.
- There were certain instances where there are no annotations available in the ground truth files and our algorithm while testing was giving actual cars as false positives that were leading to a downgrade in the overall precision score.
By taking into account the above pointers, and with careful analysis and refinement of the existing methodology, it is possible to make targeted adjustments that will result in a notable improvement in the model’s precision performance.
8. Result
These demo satellite images provide a glimpse into the power of our trained models, as they detect aerial objects with precision and accuracy. With each image, our trained models reaffirm their ability to identify and track aerial entities, underscoring their potential in various fields, from surveillance and security to environmental monitoring and beyond.
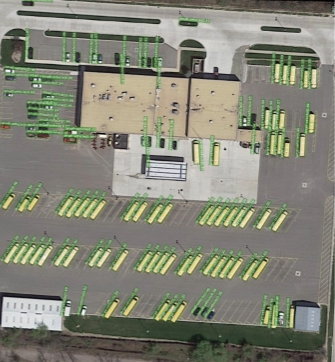
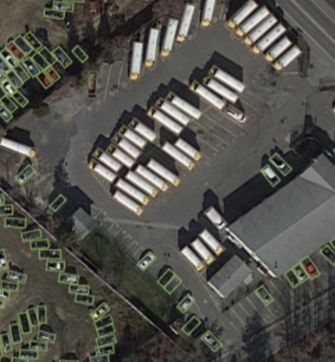
Fig 22: Demo satellite images
9. Applications
We conducted an extensive survey of existing methods for aerial object detection and trained state-of-the-art models by fine-tuning various hyperparameters. The ultimate objective of our work is to explore the potential applications and benefits of incorporating aerial object detection into different domains. By expanding the capabilities of this technology, it can be seamlessly integrated into the following applications:

Surveillance
The integration of aerial object detection into CCTV camera recordings has the potential to significantly enhance security measures by analyzing the captured footage to identify suspicious or unauthorized aerial objects.

Agriculture
Aerial detection holds immense potential for analyzing crops in vast agricultural areas. With the ability to capture high-resolution images from above, this technology can assist farmers in monitoring crop health, identifying areas affected by pests, and optimizing irrigation and fertilization practices.

Traffic analysis
Analyzing traffic patterns from an aerial perspective can give transportation authorities valuable insights into congestion, traffic flow optimization, and accident prevention.

Forestry
Satellite/Drone image analysis can be used for forest protection, monitoring of illegal logging or global forest supply, calculating forest areas and their growth, preventive measures against wildfires, and protecting rivers.

Urban planning/real estate
Aerial models can be helpful in identifying potential areas for urban planning, allowing for efficient allocation of resources and infrastructure development.
Conclusion
Object detection in aerial images presents unique challenges, such as arbitrary orientations, scale variations, and large aspect ratios, however, the recent advancements in deep learning have contributed to significant breakthroughs in this field. The availability of large-scale datasets like DOTA has facilitated the development of state-of-the-art models for aerial object detection. By employing techniques like oriented bounding box annotation, rotation-invariant layers, oriented response networks, and feature pyramids, notable results have been achieved in aerial object detection accuracy. In conclusion, the applications of aerial object detection are wide-ranging, encompassing areas such as surveillance, agriculture, traffic analysis, and urban planning, where it can contribute to improved security, crop analysis, traffic management, and urban development respectively.
About the author
Dipayan Mukhopadhyay is an Associate Lead Data Scientist at Sigmoid. He has over 6 years of experience in Data Science, Statistical Modelling, Computer Vision, and NLP. With his extensive knowledge and experience in Data Science projects, he helps enterprises in Retail, CPG and Manufacturing extract meaningful insights from data to drive informed decision-making.