How a modern data architecture on the cloud ensures data quality and data security for banks
Reading Time: 6 minutes
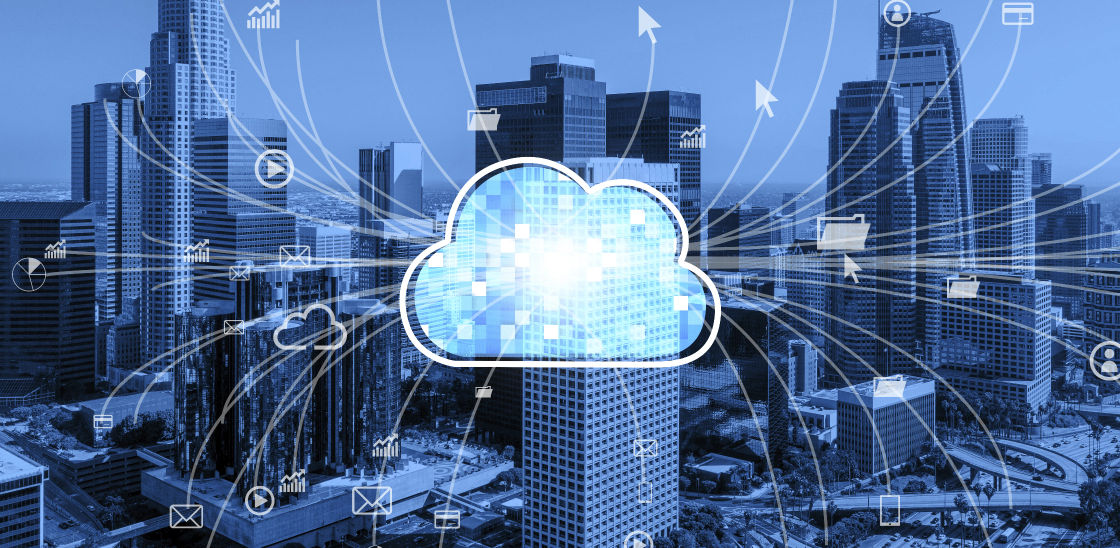
In today’s digital economy, data plays a major role in giving banks a competitive edge. Banks today should be agile, secure, responsive, efficient, and collaborative. From customer information to financial transactions, data is what allows banks to make informed decisions and operate effectively. But with the rise of cyber threats and regulatory scrutiny, data quality and security have become critical concerns for banks.
According to a study by IBM Security, the average cost of a data breach for financial services companies is $5.85 million, with the average cost per lost or stolen record being $245. Additionally, the study found that it takes an average of 233 days to identify and contain a data breach, which can have severe financial and reputational consequences for banks.
To address these challenges, banks must embrace a modern data architecture that leverages cloud computing and helps banks consolidate and integrate their data, while also ensuring that it is accurate, consistent, and secure. In this blog, we’ll explore how a modern data architecture on the cloud can help banks achieve unparalleled levels of data quality and security.
Challenges that affect data quality in today’s banks
Banks face several challenges when it comes to ensuring high levels of data quality. Modern data architecture can tackle these challenges by providing robust data governance and security measures. A report by McKinsey & Company found that banks that invest in data quality initiatives can achieve a 20-30% reduction in data processing costs. Some of the most pressing challenges related to data quality are:
1. Data silos
Banks store their data in multiple systems, departments, and formats, leading to data silos. This makes it difficult to integrate data and analyze it effectively and can create data redundancy, inconsistency, and inaccuracy. This also results in higher operational costs, longer turnaround times for data requests, and missed opportunities to leverage data for business insights.
2. Data inconsistency
Storing data with varying formats or naming conventions can cause discrepancies in banking data such as customer information or transaction details. This can lead to difficulties in identifying and tracking customers, processing transactions, and making informed business decisions. It can also result in compliance issues with regulators, leading to hefty fines and reputational damage.
3. Lack of data governance
Banks need to ensure that their data is accurate, complete, up-to-date, and compliant with regulatory requirements. Poor data governance practices, including lack of data ownership, inadequate data standards, and insufficient data lineage, can hinder effective risk assessment, fraud detection, and operational resilience.
4. Data gaps
Lack of reliable data, including missing values or data gaps, can hinder data integration efforts which limit a comprehensive view of banking operations and customers. It can impact critical areas such as risk assessment, fraud detection, and transaction processing.
A modern data architecture on the cloud can help banks overcome these data quality challenges and mitigate reputational damage. One of the key benefits of cloud-based data architectures is the ability to automate data quality checks, which ensures data consistency and accuracy across all sources. This involves detecting and correcting data errors in real-time, flagging data inconsistencies, and alerting the relevant stakeholders. They also provide better data governance capabilities by enabling banks to track and manage data lineage, metadata, and access control policies. By having a unified view of data across the organization, banks can establish clear data ownership, manage data usage permissions, and ensure that data is accurate and up-to-date.
In terms of real-world use cases, banks have already begun leveraging modern data architecture to improve their data quality. For example, Goldman Sachs has implemented a cloud-based data lake to enable real-time data processing and analysis, while JPMorgan Chase has developed a centralized data management platform to streamline data governance and improve data quality.
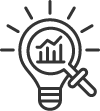
Leverage data quality management for tactical decision-making and operational efficiency.
Enabling quality data on the cloud
By integrating cutting-edge technologies and leveraging the scalability provided by a cloud-based data architecture banks can establish a solid foundation for data quality initiatives. It provides a dynamic and agile environment that fosters efficient data integration, validation, cleansing, and governance. Here are the key activities to take into account:
1. Data validation and cleansing
Implement data validation rules and checks to identify and eliminate errors, inconsistencies, and outliers in the data. Perform profiling to understand and discover data, gain insights into what measures of quality can be applied, and identify areas that require improvement. Cloud-based data integration services like Azure Data Factory provide data validation and cleansing capabilities through its data flows feature.
2. Data integration and governance
Ensure that data integration processes adhere to industry standards and best practices, such as data lineage tracking and metadata management. Implement data governance practices to define data ownership, accountability, and access controls. Google Cloud Data Fusion is an example of a cloud-native data integration service that accelerates the process of building and managing data pipelines with integrated data validation module for data cleaning.
3. Data monitoring
Set up automated monitoring and alerting mechanisms to identify data quality issues in real time. Additionally, regularly analyze data quality reports to identify trends, patterns, and potential areas of improvement. AWS CloudWatch provides real-time monitoring and alerting capabilities to track data quality metrics and detect anomalies.
Securing banking data on the cloud
Banks have a critical need for data privacy and security, and cloud-based data architectures can protect against unauthorized access, cyber-attacks, and other threats. It offers robust security measures such as firewalls, intrusion detection systems, and Security Information and Event Management (SIEM) tools to protect against such threats. Here are some of the key security features of a modern data architecture:
1. Data encryption
It is the process of converting plain data into encoded data, making it unreadable for anyone without the proper decryption key. It is a powerful tool to secure data while it is in transit or at rest. Cloud-based data architectures provide robust encryption options, for example, Amazon Web Services (AWS) offers multiple encryption options, including server-side encryption, client-side encryption, and encryption of data in transit with Secure Sockets Layer (SSL)/Transport Layer Security (TLS) protocols.
2. Access controls
Banks can set specific permissions and access levels for different users, ensuring that only authorized individuals have access to sensitive data. With cloud-based data architectures, banks can easily manage user roles and permissions, and revoke access when necessary. For example, Microsoft Azure offers Azure Active Directory, which provides identity and access management for cloud-based applications, including access control and single sign-on capabilities.
3. Data backup and recovery
It ensures that data is recoverable during a data loss or system failure. Modern data architectures typically provide automated backup and recovery features, allowing banks to recover their data quickly and with minimal data loss. Cloud-based services such as Amazon S3 versioning and Microsoft Azure Backup allow banks to recover their data quickly and with minimal data loss.
4. Data privacy and anonymization
Data anonymization techniques are increasingly used by companies to protect sensitive data such as Personally Identifiable Information (PII) and safeguard privacy. Techniques like data masking, hashing, encryption, replacement/substitution, scrambling, and blurring are generally used for data anonymization. Additionally, banks must adhere to directives such as the General Data Protection Regulation (GDPR) and the California Consumer Privacy Act (CCPA) to protect sensitive customer information. Data privacy and anonymization are essential for fostering customer trust, maintaining brand reputation, and complying with legal and ethical standards.
5. Data compliance
Banks are subjected to various compliance requirements to ensure they operate within legal and regulatory frameworks. Cloud-based data architecture offers significant advantages in helping banks achieve and maintain compliance with various regulations such as Anti-Money Laundering (AML), Markets in Financial Instruments Directive (MiFID II), Sarbanes-Oxley Act (SOX), Securities and Exchange Commission (SEC) regulations, and Know Your Customer (KYC). Tools such as AWS Config or Azure Policy allow organizations to define and enforce data policies, ensuring adherence to regulatory requirements.
Conclusion
As the banking industry continues to evolve, data quality and security have become critical concerns. A modern data architecture on the cloud can help banks consolidate and integrate their data while also ensuring that it is accurate, consistent, and secure. By embracing cloud-based data architectures, banks can automate data quality checks, ensure better data governance capabilities, and implement robust security measures to protect against unauthorized access, cyber-attacks, and other threats. As the banking industry continues to adopt these modern processes to manage data, we can expect to see unprecedented levels of data quality and security, allowing banks to make informed decisions, operate effectively, and provide their customers with the highest level of service.
Featured blogs
Subscribe to get latest insights
Talk to our experts
Get the best ROI with Sigmoid’s services in data engineering and AI
Featured blogs
Talk to our experts
Get the best ROI with Sigmoid’s services in data engineering and AI