Data Quality Management
- Chapter- 1What is data quality management?
- Chapter- 2Why do we need strategic data quality management?
- Chapter- 3What are the key components of a DQM framework?
- Chapter- 4How to implement data quality management successfully?
- Chapter- 5What are the important KPIs for measuring data quality?
- Chapter- 6Trends in Data Quality Management
- Chapter- 7Further Reads
- Chapter- 8FAQ
What is data quality management?
Data quality management (DQM) is a set of practices to detect, understand, prevent, address, and enhance data to support effective decision-making and governance in all business processes. These practices help to gain insights into data health by utilizing diverse processes and technologies on larger and more complex datasets. Effective DQM is a continuous process as data quality is pivotal for extracting actionable and accurate insights from your data.
Why do we need strategic data quality management?
Data quality management is vital for making sense of your data, and ultimately improving the bottom-line. Here are some reasons to practice DQM across your organization:
- To provide for and enforce data quality and management rules across departments, while ensuring high-quality information at every step
- Reduces costs, prevents errors and oversights by providing a solid information foundation for understanding and controlling expenses
- For meeting compliance and risk objectives, implementing policy at the database level within a framework of clear procedures and communication
- For visualizing overall data health with the help of graphs and BI reports facilitating functional and tactical decision-making
What are the key components of a DQM framework?
A DQM framework is a systematic process that consistently monitors data quality, implements a variety of data quality processes, and ensures that the data is consistent as per organizational needs.
Data profiling | Data cleansing and validation | Data monitoring | Data governance | Master data management |
---|---|---|---|---|
Data profiling involves scrutinizing your existing data to understand its structure, irregularities, and identifying anomalies in the data. | Data cleansing involves the correction or removal of detected errors and discrepancies in the data sets to improve its accuracy and reliability. | Data monitoring is the continuous process of ensuring your data long term consistency and reliablity. | Data governance involves policy creation, role assignment, and ongoing compliance monitoring to ensure that data is handled in a secure manner. | MDM is a collection of best practices for data management – involving data integration, data quality, and data governance. |
Specialized tools provide insights into what measures of quality can be applied, and identify areas that require improvement. | Various methods like machine learning algorithms, rule-based systems, and manual curation are employed to clean data. | Advanced monitoring systems can even detect anomalies in real time, triggering alerts for further investigation. | Well defined policies can guide how data should be collected, stored, accessed, and used, thereby maintaining data quality. | Effective MDM involves consolidating data from various sources to provide a unified, accurate version of key data entities, while improving data quality and process management efforts. |
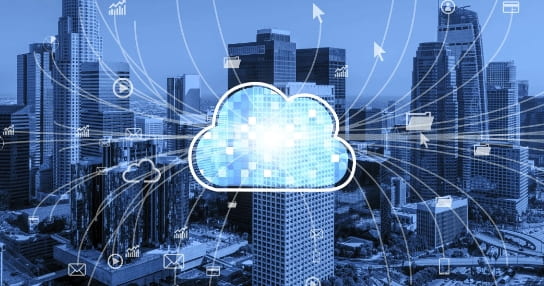
Ensuring data quality and data security for banks
With the rise of cyber threats and regulatory scrutiny, data quality and security have become critical concerns for banks. Modern data architecture on the cloud can help banks achieve unparalleled levels of data quality and security.
How to implement data quality management successfully?
A continuous process of assessment of data quality, implementation of rules, data remediation and learning feedback is necessary to run a successful DQM initiative. By focusing on key areas that are prone to data quality issues and aligning them with business objectives, organizations can significantly improve their data quality.
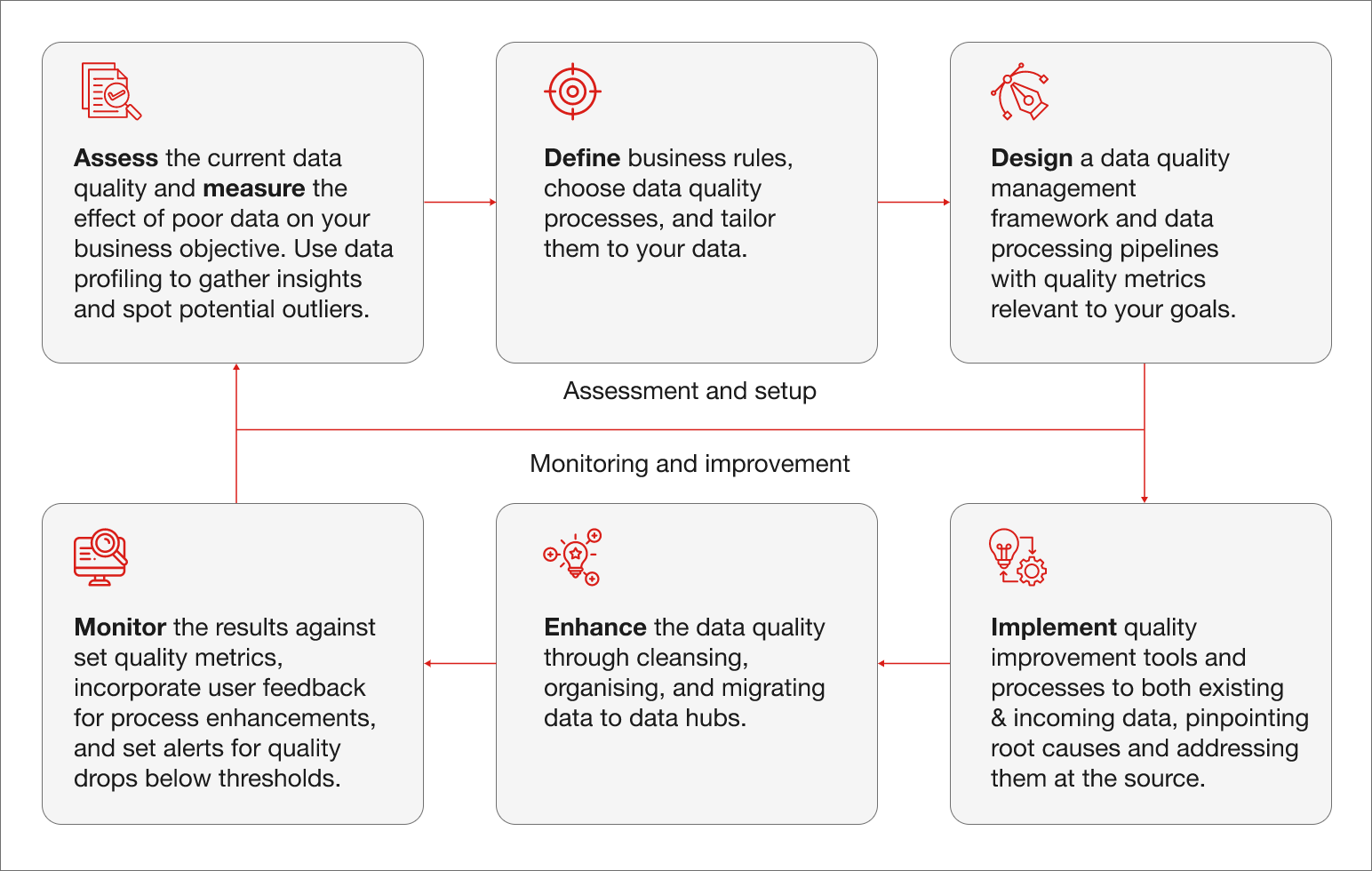
Prioritizing data quality helps businesses improve decision-making, customer experience, and operational efficiency.
Best practices for maximizing data quality:
- Assess current stage: Identify what data exists, where it’s stored, and what its current quality is. Review existing tools, data sources, types, flows, and any past attempts at data quality management.
- Define objectives and metrics: Define specific goals and objectives for data quality improvement. Determine how these objectives align with your business goals and ROI expectations. Set up metrics that offer tangible indicators to gauge the effectiveness of the framework.
- Establish a data governance guideline: Create a formal data governance framework that outlines the roles, responsibilities, and procedures for data management within the organization. Identify stakeholders accountable for different aspects of data quality.
- Implement data quality tools: Use advanced data quality tools that offer features to improve quality processes like data validation, cleansing, and profiling. Invest in tools that help automate data profiling, cleansing, data matching and validation.
- Develop a phased implementation plan: Craft a project roadmap with phases so that each phase can focus on specific objectives, much like how data cleansing targets specific data issues identified in profiling. Specify what will be done, by whom, and by when with specific milestones and success metrics.
- Create training and awareness programs: Train your employees on the importance of data quality and how to maintain it with the available technologies. Encourage a data-centric culture where everyone takes responsibility for data quality and follows through the policy.
- Perform data audits: Conduct regular audits to assess the current state of data quality. This includes identifying inaccuracies, inconsistencies, and redundancies. Use these insights to pinpoint areas that need immediate attention. Frequently monitor the results and measure against the KPIs for measuring the data quality. Any deficiencies will provide a starting point for the next round of planned improvements.
What are the important KPIs for measuring data quality?
Here are a few factors that help to determine the quality of a dataset that signify the effectiveness of a DQM implemented successfully.
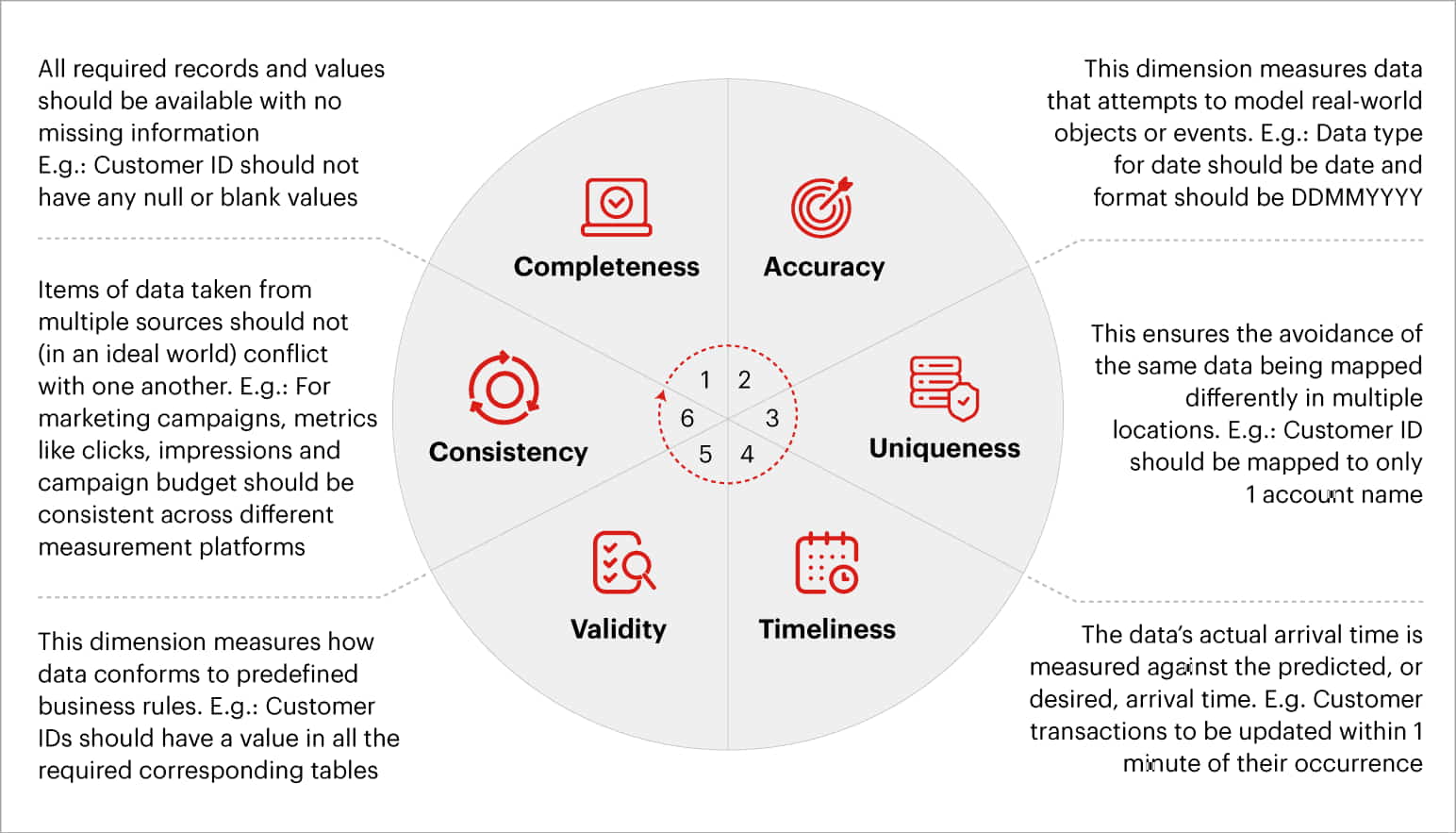
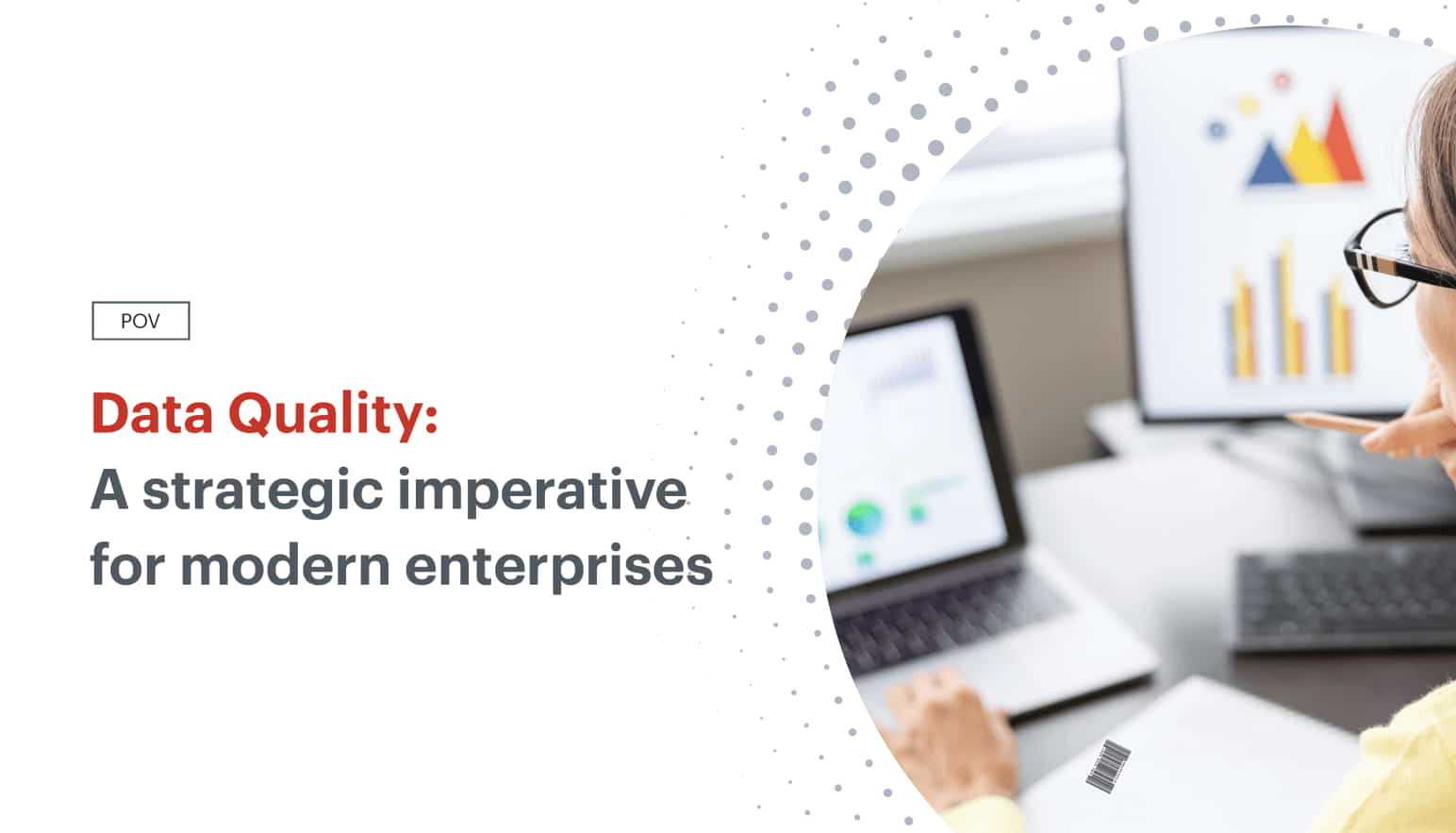
Transforming data into a valuable asset
Studies reveal inconsistent data quality can have serious implications for businesses. Maintaining high data quality across the enterprise comes with certain challenges but a holistic framework can help you close the gaps.
Trends in Data Quality Management
- Augmented data quality: This is an emerging approach that implements advanced AI techniques or ML algorithms to automate data quality management. Capabilities like metadata analytics, knowledge graphs, ML-driven rule recommendations etc. can enhance real-time data quality processing on streaming or synthetic data. This results in improved data accuracy, prescriptive suggestions, faster prediction of anomalies or discrepancies and automation of process workflows for rapid time to value with a reduced operational cost.
- Data warehouse modernization: By adopting modern data warehousing solutions, organizations are better equipped to maintain and enhance data quality. These modern platforms often incorporate data quality features and capabilities, making it easier to identify and rectify data issues before they propagate throughout the organization.
- Cloud-native DQM tools: Cloud-based data quality tools provide scalability, flexibility, and accessibility that were previously unattainable with on-premises solutions. By leveraging cloud/hybrid cloud data technology, organizations can centralize their data quality processes, ensuring that data is consistently validated and cleansed as it flows through various systems and services.
- Rise of data hubs: The growth in demand for structured data warehousing and management has led to a wide array of modern data hubs that offer various balances of advanced tools. These hubs keep data moving and provide a holistic approach to data management, all the way from curation to orchestration.
- Data quality as a self-service capability: Businesses are moving towards a self-service model for data quality management. This means that business users will be able to identify and fix data quality issues on their own, without having to rely on IT support.
Further Reads
Data quality management success stories
FAQs
Data quality management offers a host of benefits such as:
- High-quality data provides a more accurate and complete view of the business, leading to better insights and more informed decisions.
- Clean and consistent data enhances operational efficiency by reducing errors and redundancies. When data is accurate, employees can perform their tasks more effectively, leading to streamlined operations, lower operational costs, and increased productivity.
- Accurate customer data is vital for building and retaining trust. Customer information enables targeted and relevant communication, leading to improved customer satisfaction and loyalty.
- Data quality helps to comply with legal regulations, and mitigating risks associated with inaccurate or incomplete data.
Data quality tools are processes and technologies used to identify, understand, and fix errors in data. These tools help in maintaining high data quality and support effective governance for operational business processes and decision-making. There are some packaged tools available that include critical functions like profiling, parsing, standardization, cleansing, matching, enrichment and monitoring.
Data quality control is a process to maintain the accuracy and consistency of data across the organization. It involves application of methods or processes to make sure the acquired/processed data meets overall quality standards and criteria for all values.
A data quality manager would be responsible for making sure that the data collected by the organization is compliant with a set of standards laid out by the organization. Data quality manager is also called a data steward in many organizations, responsible for the administration and supervision of an organization's data assets, and supports the delivery of high-quality, consistently accessible data to business users.