Role of AI/ML in enhancing overall equipment effectiveness for Industry 4.0
Reading Time: 5 minutes
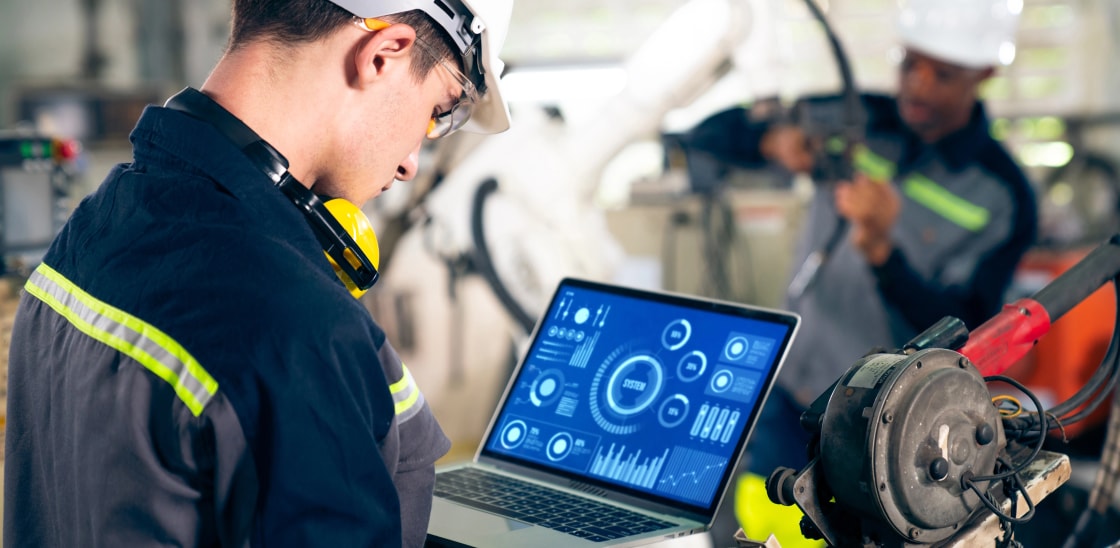
The manufacturing industry has historically been a hotbed of innovation. And, manufacturers have always pursued greater speed, scale, and simplicity across operations to optimize production, while enhancing efficiency and reducing costs. Today, the competition in the manufacturing space is mostly against time and ensuring customer engagement and meeting customer expectations regarding product quality.
Competing to thrive in a dynamic market landscape and growing quicker than competitors require manufacturers to harness real-time data for quick decision-making. The good news is that today, in the era of Industry 4.0, manufacturers have the opportunity to harvest large streams of process and production data in real-time to optimize quality, enhance efficiency, reduce equipment downtime and curb costs.
With technologies like AI, IIoT, ML and analytics, companies can create a hyper-connected manufacturing ecosystem underpinned by data to constantly optimize critical efficiency-related KPIs like OEE (Overall Equipment Effectiveness). This can help accurately identify, predict, and prevent unplanned equipment failure downtime and quality issues.
OEE as a Manufacturing KPI – Where’s the Value?
The need to reduce waste and enhance process efficiency has long compelled manufacturers to leverage OEE as a performance benchmarking metric. OEE combines three key indicators – equipment availability, throughput, and quality- to provide an accurate evaluation of particular equipment or production line performance.

OEE can be considered as a set of “best practices” metrics that evaluate the overall production time that can be counted as tangibly productive. A 100% OEE score means perfect production with minimal wastage and no downtime.
It’s important to note that companies do not essentially need to harness real-time data to calculate OEE, since the equation came into the picture in the late 1960’s – long before the advent of cloud computing or IoT sensors. But the value of OEE as a metric becomes even more impactful when real-time data is brought into the equation. It can help companies derive a more clear and detailed observation on OEE. For instance, floor managers in plants can monitor daily, weekly or seasonal fluctuations in production and equipment effectiveness with real-time data collection and analysis to avoid equipment failure.
Increasing the Efficacy of OEE as a Metric – The Need for a Robust Data Infrastructure
Collating equipment data is now easier than ever, thanks to the ubiquity of smart sensors, temperature monitors and remote sensing devices that can feed data in real-time to an OEE monitoring platform or OEE software for plant managers to evaluate. Since sensors are no longer tethered to a physical network and can be small enough to be embedded on equipment or within a product as well, data collation for OEE and monitoring efficiency is no longer confined within the four walls of a manufacturing plant. Using IIoT sensors on the equipment, any organization can effectively track the effectiveness by collecting data and analyzing availability and quality metrics from anywhere, anytime.
However, the quality and effectiveness of the OEE insights generated depend a lot on the kind of data infrastructure a company maintains. Siloed processes across multiple functions often serve as a barrier to data integration and processing, resulting in reduced analytics effectiveness and slow decision making. An effective real-time OEE platform needs to be seamlessly connected to all the functions and stakeholders involved in the production process. It should also standardize data collection and processing methodologies, terminologies and reporting procedures across all sites to facilitate fast and effective decision-making.
Companies need to have an effective data engineering process in place in order to derive rich insights on manufacturing performance out of the collated OEE data. With robust data governance and a continuously monitored data pipeline, companies can drive small yet significant improvements in the OEE scores, which can eventually transpire into substantial enhancements in profitability and efficiency.
Leveraging AI and ML to Catalyze OEE
Real-time data can serve as a powerful catalyst driving sustainable manufacturing growth from the shop floor to the board rooms. By leveraging powerful AI and ML engines, companies can derive meaningful OEE insights that not only enhances decision-making but also help companies meet a diverse set of requirements across the manufacturing value chain, such as:
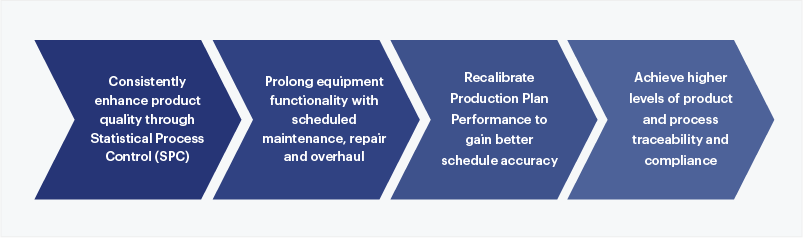
1. Consistently enhance product quality through Statistical Process Control (SPC)
AI and ML can analyze OEE data to classify production lines and plants operating at the highest capacity and the ones that aren’t. Plant operators can leverage AI to set up automated alerts within the SPC modules to notify quality management and production engineering teams of a notable deviation in equipment performance. This can help companies avert millions of dollars in lost production time.
2. Prolong equipment functionality with scheduled maintenance, repair and overhaul
By combining machine learning with predictive analytics, plant operators can get actionable and prescriptive insights into how the life span of production equipment can be extended. Long-standing theories around preventive equipment maintenance are fast changing due to the increased prevalence of real-time data. Today, companies have the opportunity to leverage intelligent systems to figure out when a piece of equipment will need a repair or overhaul.
3. Recalibrate Production Plan Performance to gain better schedule accuracy
Even a few years back, pure assumptions on fixed production times often held back an entire production line from achieving more. Today, companies have the liberty to leverage AI to fine-tune production time on Bill Of Materials (BOM) and review the same for accuracy. Accurate scheduling based on actionable insights from the plant floor can help manufacturers make production plans more agile, enhance productivity and drive proper machine utilization.
4. Achieve higher levels of product and process traceability and compliance
Innovations around PLC-based monitoring tools and Machine to Machine (M2M) interfaces have made it possible for companies today to capture real-time data on critical metrics and KPIs. For instance, companies can capture item numbers, details of work order, manufacturing date and time in one consolidated dashboard. This makes traceability one of the strongest aspects of modern manufacturing operations.
Going forward, as technologies like IIOT, AI and ML become more ingrained in the manufacturing ecosystem, operations managers across factory floors are expected to achieve unprecedented levels of equipment effectiveness while delivering more better quality products. But the road towards that future starts with building a strong data core which will ultimately dictate the success that a process achieves. The time to harness data as a competitive advantage is now.
About the Author
Bhaskar Ammu is a Senior Data Scientist at Sigmoid. He specializes in designing data science solutions for clients, building database architectures, and managing projects and teams.
Featured blogs
Subscribe to get latest insights
Talk to our experts
Get the best ROI with Sigmoid’s services in data engineering and AI
Featured blogs
Talk to our experts
Get the best ROI with Sigmoid’s services in data engineering and AI