5 ways IoT-based predictive maintenance generates business value
Reading Time: 4 minutes
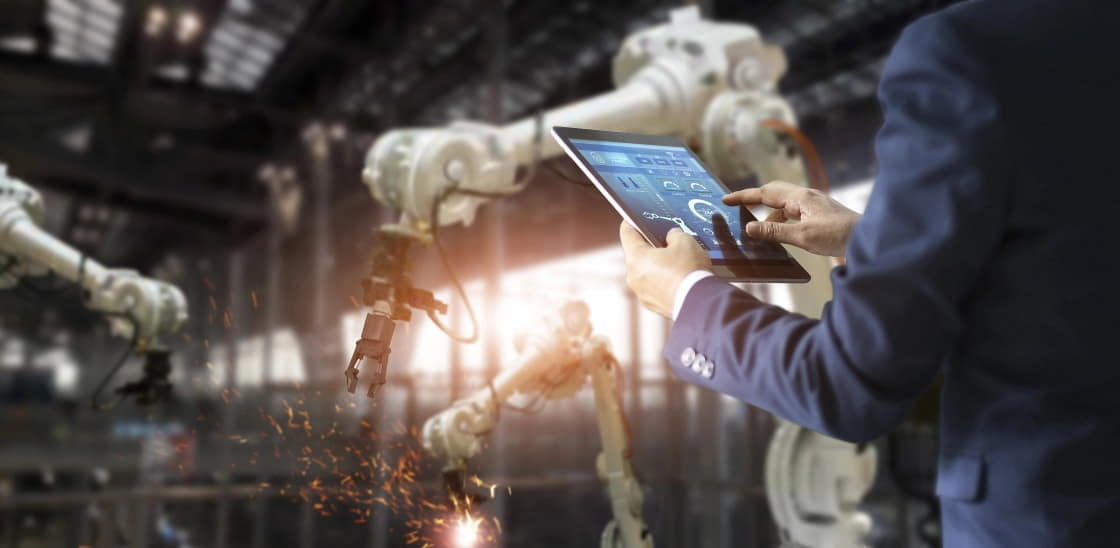
The ability to predict faults and address them before they reach critical condition is indispensable, and being able to perform maintenance while equipment is functional also cuts down on any interruptions to operations. Recent research has shown that unplanned downtime costs the industrial sector an estimated $50 billion every year. IoT and AI-driven analytics have transformed how companies approach equipment maintenance and production line management. With sensors capable of collecting real-time data across a variety of devices, assets, systems, and locations, IoT-based predictive maintenance enables organizations to effectively anticipate and plan for events such as part replacements or equipment breakdown.
Below are 5 key benefits of IoT driven predictive maintenance:
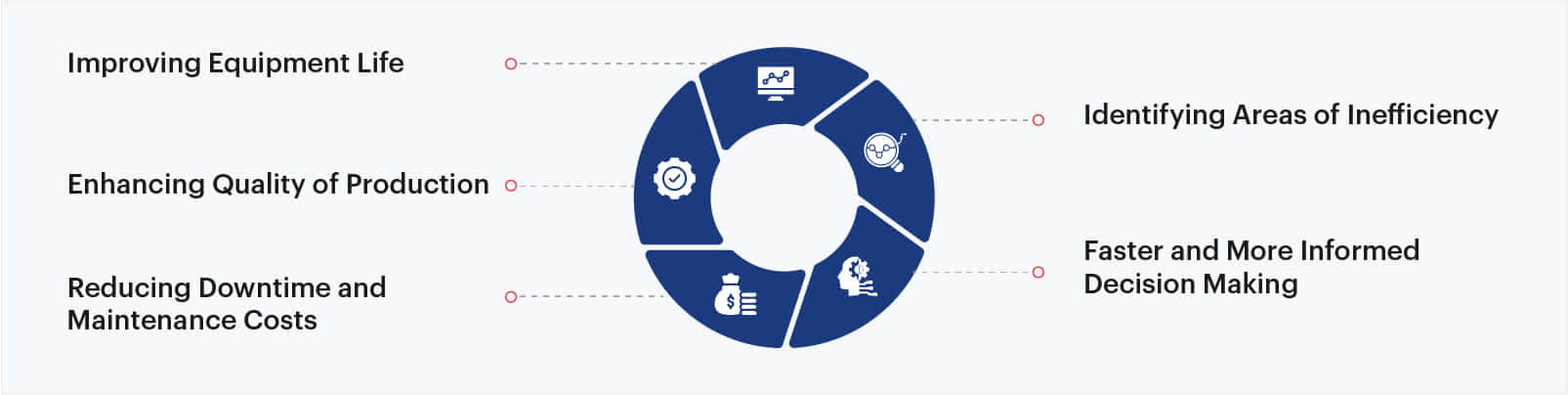
1. Improving Equipment Life
While a mix of quantitative and qualitative metrics have been traditionally used to forecast problems to reduce downtime, predictive maintenance can allow companies to successfully maximize equipment life. Real-time equipment and production-line data generated by IIoT sensors can provide companies with a comprehensive view of equipment health to effectively prioritize and schedule repairs, as well as optimize them for better performance. By predicting potential failures before they occur, teams can address any issue before it reaches the point of no return and can keep machinery and devices running at peak efficiency for longer timeframes.
2. Enhancing Quality of Production
According to a recent study, the industrial sector highlighted how predictive maintenance implementations yielded a positive ROI in 83% of the cases and that 45% of those surveyed reported amortization in less than a year. This is due to the ability of predictive maintenance systems to detect errors in real-time. When issues can be dealt with proactively, factors that affect overall productivity such as downtime, costs, and safety issues will no longer hamper businesses as much as before. Maintenance teams can achieve peak efficiency by preemptively scheduling repairs, instead of being reallocated to malfunctioning machinery. With IoT systems providing constant data and changes to processes on a regular and timely basis, problems can be dealt with while at the same time improving overall production quality.
3. Reducing Downtime and Maintenance Costs
In this regard, the evolving state of IoT-based predictive maintenance will allow operators to be more proactive when it comes to identifying equipment faults. For equipment-driven industries, in particular, historical data gathered from a variety of IoT devices and sensors can provide key metrics on machine health, usage, and risk areas. In the absence of complete historical data, predictive AI-powered models can help create frameworks that fill in the gaps by simulating real-world use cases. When action is planned around the data gathered, it can cut down on costs greatly while also heavily reducing downtime.
4. Identifying Areas of Inefficiency
Sensor data analytics and advanced algorithms can more accurately generate leads when it comes to equipment. Quick, real-time insights are crucial for spotting inefficiencies early on, and can even be used to improve systems as needed. For example, the data insights from an IoT-based sensory framework that is complemented by AI solutions can open new avenues for a power company to improve its production grid and gain greater benefits. Furthermore, potential safety risks, hazardous situations, and compliance issues can be spotted and appropriate mitigation can be done.
5. Faster and More Informed Decision Making
Due to their interconnected nature, IoT devices can function as real-time data providers anytime, anywhere. For predictive maintenance, this is the icing on the cake: a way to make quick, well-informed decisions from a location-agnostic position. Data insights that are collected by sensors and manufacturing analytics provide a clear picture of faults, improvement areas, and other key performance markers for managers to focus on as needed.
Going forward, predictive maintenance as a solution will be embedded as a standard requirement in any enterprise software. However, in order to ensure that the solution is reliable, companies will have to ensure that they have a robust data architecture to complement advanced machine learning models. After all, the number of data sources in an industrial setup is constantly increasing, and in the future competitive advantage for companies will depend a lot on nurturing strong data processing capabilities right at the point where data is being collected.
Featured blogs
Subscribe to get latest insights
Talk to our experts
Get the best ROI with Sigmoid’s services in data engineering and AI
Featured blogs
Talk to our experts
Get the best ROI with Sigmoid’s services in data engineering and AI