3 ways data engineering and real-time data analytics can boost productivity on the factory floor
Reading Time: 5 minutes
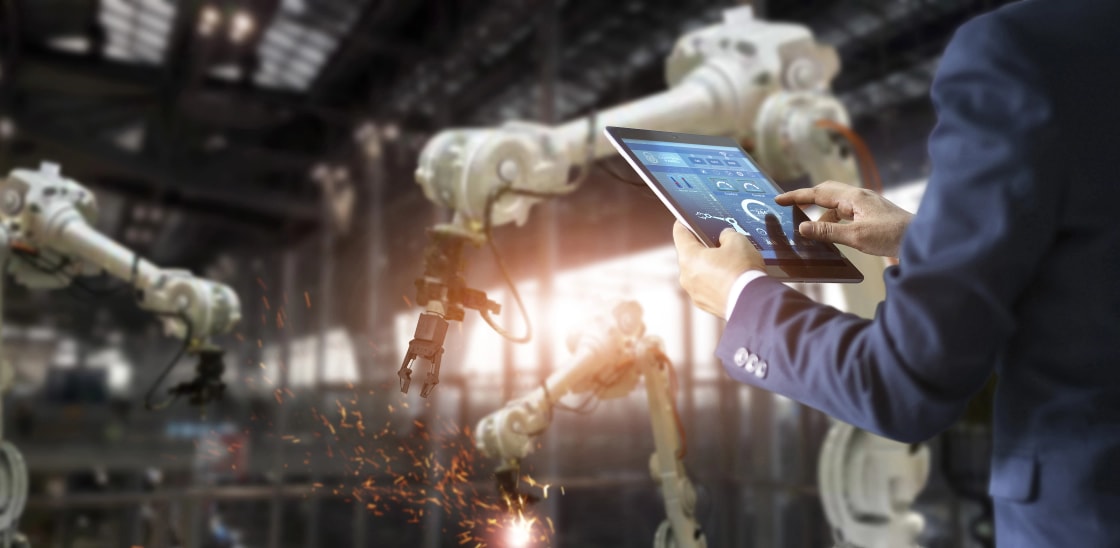
Maximizing productivity while ensuring highest product quality and sustainability has always been crucial for the manufacturing industry. Traditionally, manufacturers have relied on either manual tools for information gathering that took weeks to track data from end-to-end manufacturing processes and identify any failures. However, today’s competitive world requires manufacturers to turn to smart manufacturing to gain real-time information on their processes to optimize outputs as opposed to waiting for weeks to locate issues.
The advent of the Industrial Internet of Things (IIoT), digital thread, and AI have played a significant role in achieving production excellence in the manufacturing industry. IoT and sensor technology has made it possible to track machine performance 24/7 that enables manufacturers to establish smoother operations by minimizing the impact of unanticipated production delays. With the digital thread, manufacturers can establish a communication network that provides an integrated view of an asset throughout the manufacturing lifecycle eliminating siloed elements in the manufacturing process. To analyze the collected data, AI is leveraged to add value by providing informed decision making enabling companies to increase the efficiency of business operations, improve quality and throughput, and reduce costs.
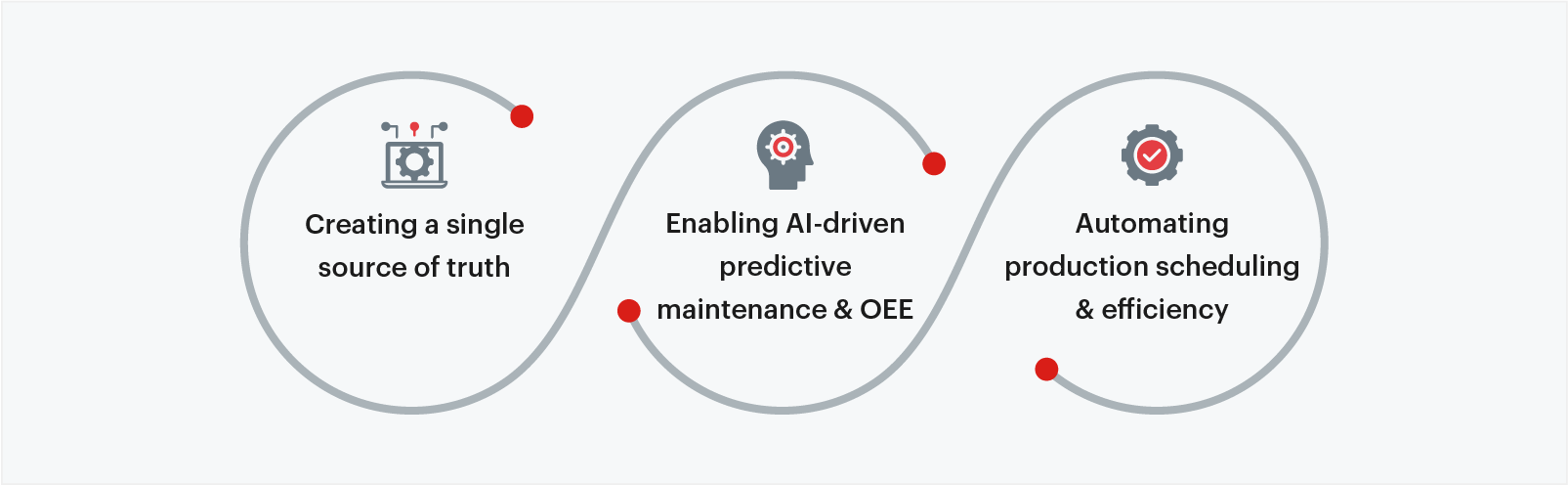
1. Creating a single source of truth
Challenge:
Data coming from multiple sources such as machines, software applications, and automation systems needs to be collated for production systems to run efficiently. However, manual processes for data extraction can lead to delays in the process along with inaccuracy or missing data. As data processing lags behind, it results in poor demand forecasting, inventory management, and scheduling decisions that can cost manufacturers millions of dollars.
Solution:
To address the challenges of inaccuracy, data silos, and unstructured data streams, IIoT and ML technologies call for a modern framework that can store the data generated by the machines and equipment on the factory floor. Unlike legacy data frameworks or databases, data lakes are designed to store unstructured or structured data as is from all types of devices. Using data lakes for data storage helps address the challenge of data silos and capitalize on real-time data analytics to improve production and enhance smart manufacturing processes. Organizations deploying data lake technology report 84 percent of information is delivered on time with higher data accuracy, according to AWS.
By connecting all the disparate data at one place, data lakes enable manufacturers to increase operational visibility and achieve optimized production with highest quality and sustainability. Manufacturers can leverage this data to improve the performance of assets and drive higher efficiency in the production process minimizing the impact of unanticipated production anomalies.
2. Enabling AI-driven predictive maintenance for OEE
Challenge:
Predictive maintenance requires more frequent data on the production assets to track their performance. It is also important to collect structured consistent data from various sources while maintaining data security. Most often, data processing is slow leading to delay in insights for effective overall equipment effectiveness (OEE).
Solution:
Data engineering can solve these challenges in predictive maintenance by building modern data infrastructure with data aggregation from various sources to ensure timely inflow of information. Importantly, it implements robust data governance frameworks to ensure data security. Data engineering tools also help streamline data processing pipelines to ensure faster processing and quicker access to insights. Gaining access to accurate asset data enables manufacturers to plan production performance and improve scheduling. Accurate scheduling based on actionable insights from the plant floor can help manufacturers make production plans more agile, enhance productivity, and drive proper machine utilization.
In addition, manufacturers can leverage AI and ML to derive meaningful insights on overall equipment effectiveness to enhance decision making and prolong equipment functionality with scheduled maintenance, repair, and overhaul. The combination of machine learning, IoT enabled sensors, and predictive analytics enables improvement in OEE. It also equips manufacturers with real-time data on assets such as details of work order, manufacturing date and more to detect wear and tear, and faults in a given machine, ahead of time and proactively schedule maintenance to avoid downtime. With insights into asset health, manufacturers can make more informed decisions, take action on issues such as equipment bottlenecks, unscheduled downtime, and drive higher efficiency. This data also allows for enhanced traceability and ensures compliance.
Sigmoid built an AI solution for a leading CPG company, that evaluated the production line data and identified inefficiencies and recommended corrective action to improve overall equipment effectiveness of machines. The solution resulted in a 2.5% improvement in OEE apart from enabling real-time alerting for machine downtime, failure, and line losses.
3. Automating production scheduling and efficiency
Challenge:
Manufacturers usually take a few days to update any change in demand, raw material availability or safety stock requirements in the schedule. Inaccurate or outdated schedules can cause unnecessary delays in the production process, which can lead to significant losses in the business.
Solution:
Building schedules with ML technology can help provide new opportunities to make intelligent decisions based on available data for smart manufacturing. By automating and optimizing production scheduling on every new change, ML models can eliminate delays in scheduling and simplify the process without disrupting the entire production process. With ML powered scheduling, manufacturers can reduce time, improve reliability, shorten setup times, reduce waste, handle exceptions in real-time, and control fixing times.
As a case in point, Sigmoid built an automated master production schedule for a Fortune 500 pharmaceutical company to increase capacity utilization to 15%, reduce the time spent on schedule generation by 98%, and offered near real-time visibility into KPIs and metrics.
Conclusion
To thrive in an ever evolving competitive landscape, manufacturers need to harness the power of data engineering and data science capabilities to leverage the value of real-time data analytics into manufacturing equipment, sales cycles, product pricing, production capacity, and more for informed decision making. These practices help manufacturers carry out internal quality audits and meet regulatory compliance requirements, which is particularly helpful for manufacturing of products catered to strict compliance requirements like medical devices. Since the flow of information is accurate and in real-time, it also increases visibility and fosters transparency across operations.Smart manufacturing processes not only benefit from reduced operational costs but also achieve high quality products leading to customer satisfaction,increase in market share and revenue.
In today’s connected world, it is imperative for manufacturers to become data driven and make decisions based on real-time data analytics to increase the efficiency of business operations, improve quality and throughput, and reduce costs. Manufacturers can also upgrade their techniques and products and decide on a future course of action based on changing market conditions and customer demands.
Featured blogs
Subscribe to get latest insights
Talk to our experts
Get the best ROI with Sigmoid’s services in data engineering and AI
Featured blogs
Talk to our experts
Get the best ROI with Sigmoid’s services in data engineering and AI