Defining the right data analytics KPIs to drive business success
Reading Time: 5 minutes
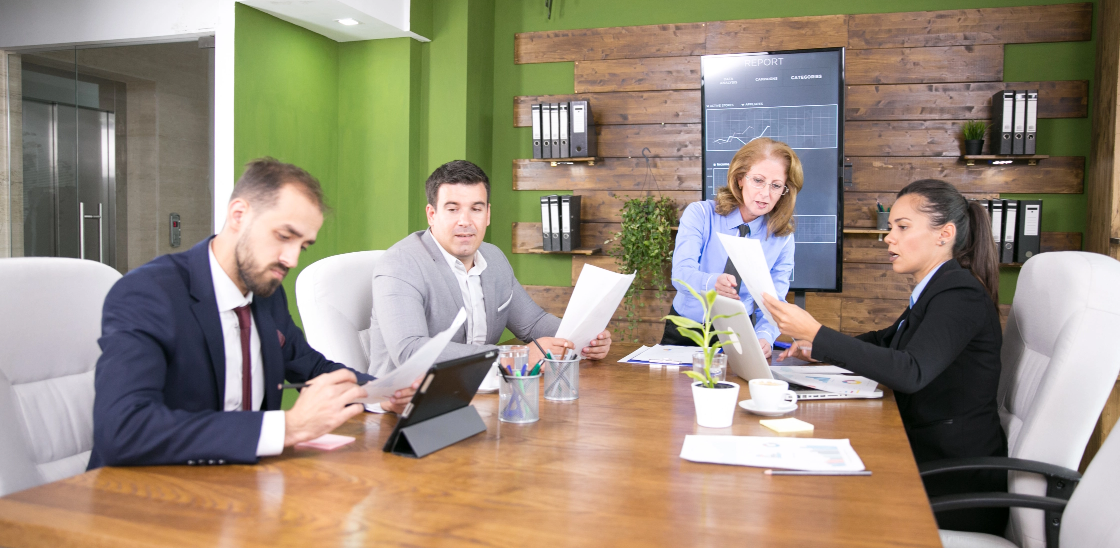
Business leaders often find it challenging to determine the right success metrics to assess the impact of Data and Analytics(D&A) programs. A Gartner report revealed that nearly 85% of D&A strategies do not mention success metrics or tangible goals. A strategic approach to data analytics helps organizations use critical insights to align with business goals and achieve desired outcomes.
Acting as a bridge between data and organizational goals, analytics KPIs help track business performance and allow business leaders to make the right decisions. Analytics KPIs essentially showcase if the organization is on the right track to achieve desired results. Giving a clear direction to business data, analytics KPIs are designed to offer relevant and valuable insights on business growth.
Evolution of KPIs
Data analytics has brought a new spin in every aspect of business, KPIs are no exception. While business KPIs were restricted to sales and revenue metrics, today they include clicks, customer visits, average user session, lead conversion and more. With a number of metrics factoring in, it is extremely crucial for organizations to stay updated with data-driven consulting. Similarly KPIs for supply chain have gone beyond order fulfillment, it includes many others such as raw material procurement, forecasting, and hedging to procure raw materials at an affordable price beforehand. Order fulfillment has expanded into identifying the best logistical methods for cost effective transportation and distribution.
With the introduction of AI models, there have been new sets of KPIs to measure the performance of these models. Analytical measurement or statistical model KPIs directly replicate business goals rather than using standard metrics to gain desired results. At the end, it is the incremental dollars that count.
The role of business consulting is only growing as the KPIs landscape is evolving by the day. Data analytics consulting workshops that involve detailed discussions with multiple business stakeholders is key to understanding expectations from business transformation initiatives and to develop an analytics roadmap for the organization. Businesses have benefited from working with data analytics companies who understand their business challenges, existing systems, and KPIs to suggest a suitable analytics solution that closely meets their needs.
Analytics KPIs to align with business goals
Organizations need to determine the goals that need to be achieved through the analytics models in the initial phases and tie it back to the analytics KPIs while tracking model performance. These goals must be highly correlated with product metrics and business goals. Typically, data analytics companies start with ROI in mind while understanding business requirements to align with analytics strategy. For instance, for a demand forecasting model, whose business goals include increasing revenue and customer retention, reducing stockouts and inventory costs the following model tuning parameters can be used:
- Weighted absolute percentage error (WAPE)
WAPE is a way to measure the accuracy of forecasts as compared to the actual outcomes for a sample. It helps organizations to investigate errors of a forecast over time and get an insight into the accuracy and responsiveness of their data models.
- BIAS
Biased estimates usually lead to incorrect forecast accuracy due to errors in the system. Bias directly governs stockouts and inventory investments. For example, if the forecast has a positive bias, it will consistently predict higher forecasts than actual sales leading to increased inventory investments. Similarly, if the forecast has negative bias, it will predict lower numbers than the actual sales leading to stockouts. A perfect scenario means there is no bias.
- BULLSEYE
This method provides a good value for important products based on certain factors. It typically shows accurate forecasts for most products.
- COVERAGE
Coverage describes the number of products that can be predicted out of the entire range a business has to offer. In the long run, the aim is to predict forecasts for 100% of its products.
Addressing data challenges
To define valuable statistical model KPIs, it is important to identify the consumers of the insights to augment the overall business performance. Gartner reports that 80% of data analytics insights fail to deliver business outcomes. The most effective analytics KPIs are the ones that closely align with organizational goals and help answer crucial business questions. Alongside formulating a clear business goal, it is essential to look into the following aspects of data:
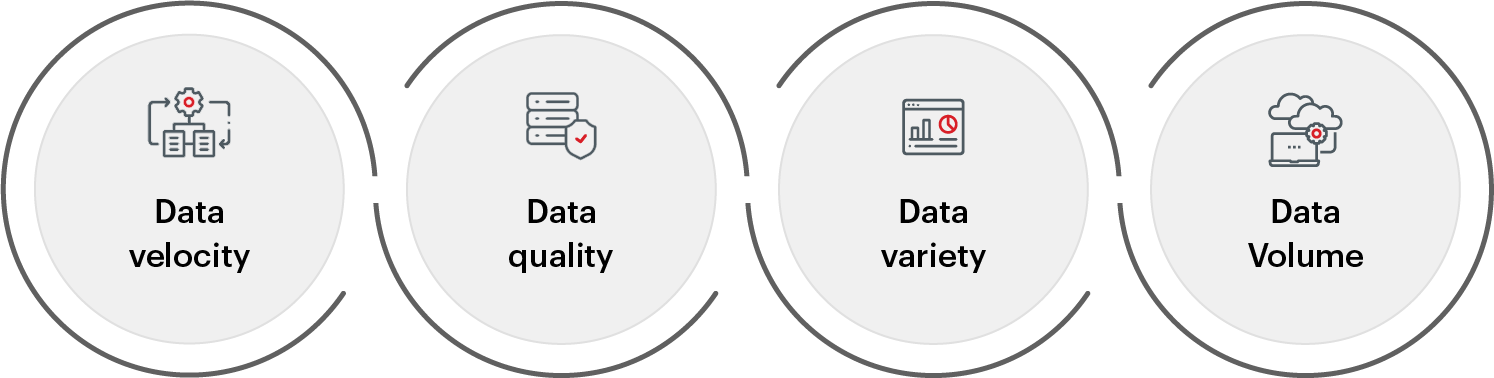
- Data velocity: Devising KPIs around data velocity to capture data and track the overall performance helps paint a clear picture of an organization’s business goals. Data analytics companies can provide near real-time data processing and decision making enabling organizations to churn out valuable insights more quickly. As users get more insights into data and analytics, the more flexibility they get to find answers through reports, queries, and dashboards.
- Data quality: An extremely essential part of defining reliable KPIs is the quality of data. However, most organizations are plagued with poor data quality. A research by Harvard Business Review points that only 3% of data in a business enterprise meets data quality standards. Having the right data governance models in place helps maintain pertinent data quality dimensions, enable decision makers to rely on insights offered through KPIs and enhance the overall decision making process. Businesses can also rely on exploratory data analysis (EDA) to perform initial investigations on data to spot anomalies using techniques like visualization.
- Data variety: Considering the different types of datasets including structured and unstructured is crucial to quantifying and measuring performance. These can also include data from texts, social media, and videos that bring the variety of data sources across the organization for devising KPIs. With a full stack real-time analytics platform in place, organizations can handle the variety of datasets coming in from various sources.
- Data Volume: Given the huge amount of data being generated each day, filtering, cleaning, and selecting the right data set is essential for KPIs. With new data pouring on a constant basis, organizations need to keep updating their metrics to stay relevant and respond to changes quickly. With best practices on data management, organizations can manage huge volumes of data coming in without any downtime or delay in processing.
Conclusion
The right analytics KPIs greatly help organizations to uncover exact business value generated by big data and its impact on increasing ROI. On a macro level, measuring the performance of analytics models is about achieving business objectives and measuring success rather than looking at big data as a monolith. Data analytics KPIs empower organizations to focus on information with the potential to make a bigger impact than getting burdened by vanity metrics, security logs, or the endless noise generated by various business devices and tools.
About the Author
Ketan Garg is an Associate Vice President – Data Analytics in Sigmoid. He has helped clients improve strategic and operational decision making using the power of data and analytics. In his current role he is working with multiple Retail and CPG clients throughout descriptive, diagnostic, predictive and prescriptive analytics.
Featured blogs
Subscribe to get latest insights
Talk to our experts
Get the best ROI with Sigmoid’s services in data engineering and AI
Featured blogs
Talk to our experts
Get the best ROI with Sigmoid’s services in data engineering and AI