Top 5 data engineering trends for 2023
Reading Time: 5 minutes
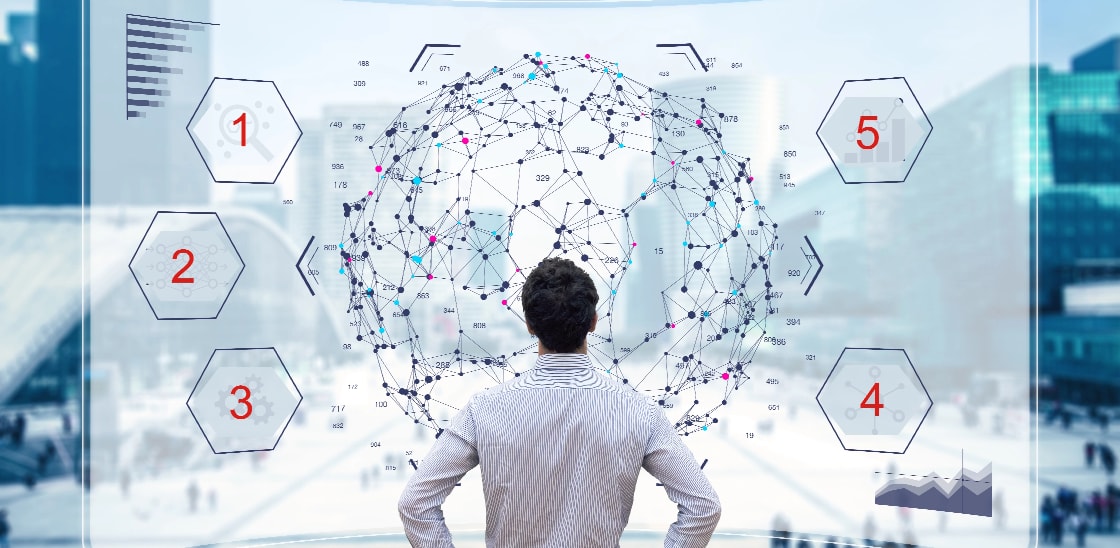
Over the past decade, Data Engineering has been enabling businesses to gain a competitive edge by providing them with insights in real time and data-driven strategies at scale. As companies are quickly moving towards being AI-driven, collecting, processing and analyzing the vast amounts of data becomes extremely important. Increased adoption of the cloud platforms and democratization of data across the organization have spurred sudden demand for data engineering skills.
Here are 5 emerging DE trends for 2023 that will attract keen attention from data professionals:
- Data Mesh establishes a new perspective to data consumption :
Data mesh is an architecture that focuses on decentralized data ownership across business functions. It embraces a new way of looking at data in an organization shifting from a single centralized data platform to multiple decentralized data repositories enabling self-service and eliminating numerous operational bottlenecks. Here are the main principles of a data mesh:
- Data as a product: As opposed to the traditional way of extracting value from data, a data mesh places value on the data as a product and enables data monetization. Data is seen as an organization’s product rather than acting as a by-product of a process. Here data producers act as product owners giving them ownership of the data.
- Self service data infrastructure: Data users across business domains can use self-service analytics tools and reporting to explore real-time insights into critical business trends improving efficiency and fostering a culture of data-driven decision making.
- Governance: Data mesh enables data to be adhered to industry standards and compliance requirements.
- Data contracts break barriers between data producers and consumers:
Typically, developers have little visibility into how the data is consumed. To that end, data consumers find it difficult to reach out to data producers to prioritize their use case creating delays and dependencies. Data contracts help eliminate these dependencies by creating API-based agreements between IT Teams and data consumers to generate high-quality, trusted, and real-time data. There are numerous data producers and multiple consumers working with data in different languages, in different databases and models. Data contracts can be designed to reflect the semantic nature of business entities, events, and attributes. This helps IT engineers to decouple their services from analytical requirements without causing production-breaking incidents while modifying databases. Data teams can focus on describing the data they need instead of attempting to stitch the world together retroactively through SQL. In a nutshell, data contracts help in:
- Increasing quality of produced data
- Maintenance of data
- Application of governance and standardization over a data platform
- Enterprises take proactive approach towards data governance
Organizations modernizing their data platform need end-to-end governance approaches in place. Data governance requires organizations to embrace technologies that lead into the scalable nature of cloud and distributed nature of data teams. This entails reframing of governance approaches across four pillars including data observability, data discovery, data security and data privacy.
- Data observability: Organizations should understand the health of the data in their system and supplement data discovery by ensuring that the data is trustworthy at all stages of the life cycle.
- Data discovery: Provides domain-specific and dynamic understanding of the data based on the way it is ingested, aggregated, and used by a set of data consumers.
- Data security: Protecting and managing modern data stack tools to restrict access to data based on roles or needs. DE teams can also partner with their security and legal counterparts to conduct data audits and comply with regulations.
- Data privacy:Ensuring that internal data processing and handling procedures are in line with regulations such as the European GDPR, CCPA, Canadian PIPEDA, and Chinese PIPL etc., In the long term, this will build trust among consumers to share their data with organizations in turn enabling businesses to develop better products.
- Feature stores improve collaboration between data teams
Features store is a centralized software library containing a number of functions or features created from standardized input data. A feature can be an independent variable affecting the prediction of an ML model. For instance, prediction of sales of a product can have a feature of stock status or competitor prices. The features can be fed into ML algorithms to solve different problems enabling data professionals to follow a common workflow for any ML use case. Acting as a data transformation service, features store enables users to sift through raw data and store it as ready to use features by any ML model. A feature store provides a single pane of glass for sharing all available features improving the collaboration between data teams. At the end, better features translate into better ML models leading to enhanced business outcomes.
Two types of features include:
- Online: The variables that undergo changes frequently and need to be updated in real-time. These features are challenging to calculate as they require faster computation and access to data For instance, weather conditions is an online feature for prediction of delivery time.
- Offline: These variables do not vary often and can be recalled through past events. These features can be calculated using frameworks such as Spark or by running SQL queries against a database. For example, order processing time is an offline feature for the prediction of delivery time of a product.
- Streaming first infrastructure for real time analytics
Streaming-first infrastructure helps solve issues of speed and efficiency by providing real-time data ingestion from the source. By streaming live events and transforming them during the process, the infrastructure synchronizes datasets and enables insights on a real-time or near real time basis while increasing scalability. For ML models that require extremely low latency and events driven message queue, streaming-first infrastructure is helpful such as fraud detection, recommendations, and personalization.
Access to real-time data enables building of ML models based on the most recent data enabling businesses to identify patterns to make informed and efficient decisions. A streaming-first infrastructure also helps achieve low frequency for downstream data consumption. Data teams can easily utilize massive data sets for ML and other AI models to improve the accuracy of predictions by adapting to new patterns and trends.
It is important to ensure that various data conglomerates of a data mesh across business domains can function seamlessly. This can be achieved with clearly defined APIs or data contracts to build trust on each other’s data products.
Conclusion
These trends are expected to have a substantial impact on data initiatives for any organization to improve operations and optimize spending. Many organizations are already adopting streaming-first infrastructure, data contracts, and feature stores to improve their AI and ML model performance. By leveraging these approaches, organizations can enhance their data and analytics capabilities while increasing ROI.
About the Author
Gunasekaran S is the director of data engineering at Sigmoid and with over 20 years of experience in consulting, system integration and big data technologies. As an advisor to customers on data strategy, he helps in the design and implementation of modern data platforms for enterprises in the Retail, CPG, BFSI and Travel domain to drive them towards becoming a data-centric organization.
Featured blogs
Subscribe to get latest insights
Talk to our experts
Get the best ROI with Sigmoid’s services in data engineering and AI
Featured blogs
Talk to our experts
Get the best ROI with Sigmoid’s services in data engineering and AI