Why is data engineering critical to CPG marketing success
Reading Time: 6 minutes
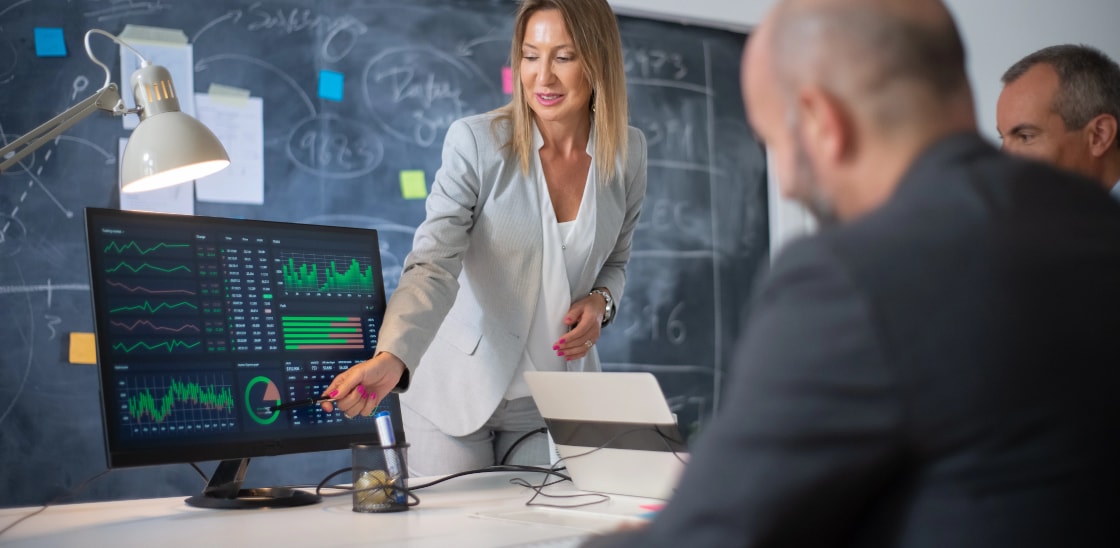
With most business processes transitioning to digital, the volume of data generated is witnessing exponential growth. Digital marketers today understand that the increase in volume and complexity of data processed on a daily basis for decision-making means that ensuring the quality & timeliness of data is critical. Research reveals that in some cases, on average companies stand to lose out $12.9 million annually due to bad data quality.
And this is where the discussion on data pipelines and their management can be pivotal. As most businesses leverage the cloud to store and process data, they need ELT pipelines that support growing analytics needs.
Challenges with Data Volume and Variety
According to a Marketing Data and Analytics survey, more than half (54%) of surveyed senior marketing leaders reported dissatisfaction with their analytics effort. Here are the primary challenges CPGs face with large and diverse marketing datasets.
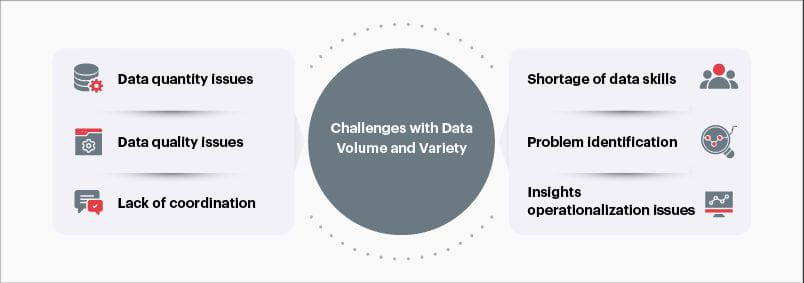
- Data quantity issues: The advent of big data in the digital era has enabled marketing teams to log every consumer action. However, marketers are grappling with structuring the huge data troves. Even to this day, experienced data scientists are spending most of their valuable time in manual wrangling and formatting data instead of analysis. This was supported by a recent survey where 41% of the respondents pointed to manual data wrangling as a significant challenge. For the CPG industry, trends like the rapid rise in online purchases, higher spending on digital media advertising, and rising Direct-to-Consumer (DTC) channels are further fuelling the situation.
- Data quality issues: Of the respondents that reported manual data wrangling to be an issue, more than half (53%) reported being unable to rely on their data due to inaccuracies. And as the Marketing Data and Analytics survey showed, poor data quality remains one of the top concerns for marketers. In fact, some researchers have pointed out that one-fourth of all businesses have lost a customer due to poor data quality. An organization must work out a process to maintain data quality so that information accuracy doesn’t hinder analytics and subsequent decision-making.
- Siloed datasets: In many cases, vast and diverse datasets are governed by a standalone business or IT teams on an ad hoc basis leading to poorly focused initiatives and misinformed decision making. This disjointed approach towards data management also leads to a lack of visibility into data assets, wrong insights generation, and increased privacy and security risks. Surveys have also highlighted a clear disconnect between data analysts and marketers. This has the potential of significant budget wastage if inaccuracies and mistakes aren’t properly communicated to marketers.
- Shortage of data skills: The Marketing Data and Analytics survey revealed that less than one-fourth (23%) of marketers prioritize skill development. Having to manually manage data quantity and quality issues are further exacerbating the issue. Along with the existing skill shortages, lack of upskilling and cross-skilling is severely hampering organizational data goals highlighting a clear disconnect between data analysts and marketers. This has the potential of significant budget wastage if inaccuracies and mistakes aren’t properly communicated to marketers.
- Problem identification: Many organizations fail to prioritize initiatives when faced with large and diverse datasets. In many cases, data initiatives fail to materialize as marketing teams prefer metrics that are simpler to track or use standard performance indicators.
- Insights operationalization issues: The fast-changing nature of the business landscape has only gained pace due to recent world events. What this means is that any data including marketing data can lose relevance rapidly. For organizations handling large and diverse datasets, it can often be a challenge to build analytics models that can be relevant and effective on ever-changing datasets.
Disparate Data Types for CPG Analytics
CPG data analytics involves the analysis of predetermined data sets both contextualized and decontextualized to generate predictions and consumer insights. The datasets include various metrics such as brand loyalty, purchase frequency, transaction information, customer demographics, distribution information, price fluctuations, competitor analysis, sales trends, or general consumer behavior information. There are several key datasets from disparate sources that CPG marketers often try to integrate to obtain a holistic view of the marketing campaigns:
- Retail data: Includes tracking and monitoring data from online retailers for a brand and its competitors. These help CPG businesses predict trends, make informed decisions, and respond rapidly to market changes.
- Marketing data: Performance insights derived from marketing campaigns across channels to optimize budgets and messaging. This allows marketers to prioritize the right channel and tactics to set efficient media bids.
- Consumer data: This dataset educates CPG companies on specific consumer journeys and their purchasing behavior. This is especially crucial since 80% of consumers prefer to purchase from brands that invest in providing personalized experiences.
While a wide variety of data sources open vast and untapped potentials in terms of insights and experience-driven decision making, it also opens up a world of potential data mismanagement which can be detrimental to the overall business goals.
Data Pipeline Best practices
So what can CPG marketers do to ensure they get the most value out of datasets that are large in volume as well as diversity? One of the best approaches is to build a modern data pipeline that is essential to data utilization. Building an ELT-based data pipeline can seamlessly integrate commercial data sources (social media, CMS, etc.) with analytics and reporting. These data pipelines have several direct benefits including cost efficiency.
Here, following a set of ELT best practices can maximize the value of collected data and boost the ROI for CPG marketers and their organizations.
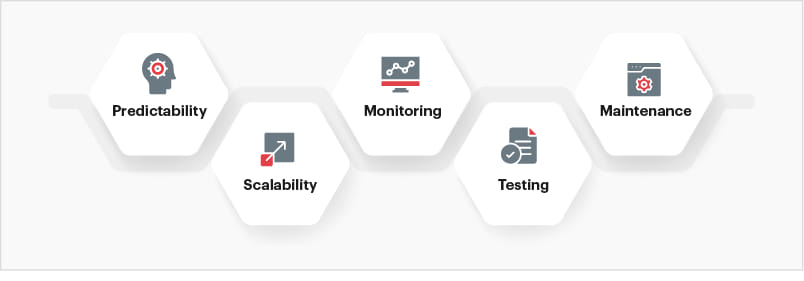
- Predictability: Eliminating unnecessary dependencies can help improve ELT pipeline predictability. This simplifies the root cause analysis for any issues as data can always be traced back to its origins.
- Scalability: Auto scaling of pipelines can help cope with changing data ingestion needs. Monitoring data volume and fluctuations can help teams establish the scalability requirements.
- Monitoring: End-to-end pipeline visibility and monitoring is critical to ensure proactive security and consistency. In the event of an issue, monitoring can enable alert triggering through real-time views and exception-based management.
- Testing: Pipeline testing helps in streamlining the system and mitigates chances of exploitable vulnerabilities. However, pipeline architecture and data testing are challenging as it includes myriad disparate processes.
- Maintenance: Pipeline maintenance should include refactoring scripts rather than augmenting dated scripts as it is not sustainable. Pipeline maintainability often depends on accuracy of records, repeatable processes, and stringent protocols.
Sigmoid Success Story
A leading oral care company wanted to create a single source of truth for all their marketing and sales data scattered across various sources and formats to enable real-time campaign optimization and analyze trends. Leveraging data engineering best practices, Sigmoid created a central repository on a cloud data warehouse, by collating and automating the ingestion of data. The data was in different formats including excel files and emails from various sources such as Nielsen, Ipsos, Lazada, Shopee, Kantar, Media & Digital Data. Sigmoid’s data science team prepared the data using BigQuery to create a single dataset and moved it into GCP for further downstream analysis and visualization using Tableau. Ten dashboards were developed for use by the marketing teams for real-time campaign reporting, optimization, and analysis. The solution reduced the time spent in creating reports by up to 45% while producing actionable insights.
Business Impact
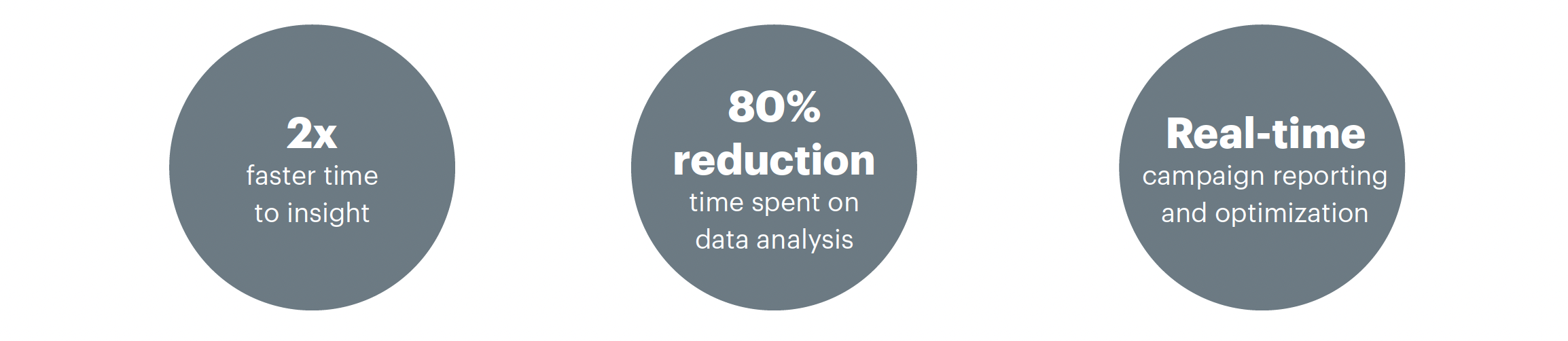
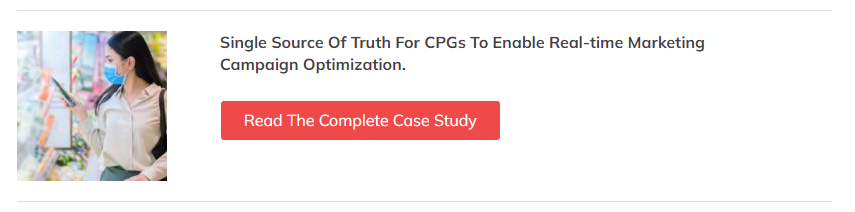
Conclusion
Like every data management principle, the best practices mentioned above provide effective guidance to extract value from a high volume of constantly changing data. As CPG marketers look for a competitive advantage using data and analytics, a robust data engineering practice that governs the availability of the right quality data at the right time to the right people becomes a necessity.
About the Author
Sudeep is a Senior Pre-Sales Manager at Sigmoid. He has a decade of experience in providing Data-driven solutions for companies across AdTech, Retail & CPG in their digital transformation journey.
Featured blogs
Subscribe to get latest insights
Talk to our experts
Get the best ROI with Sigmoid’s services in data engineering and AI
Featured blogs
Talk to our experts
Get the best ROI with Sigmoid’s services in data engineering and AI