How data-driven strategies can strengthen risk management in investment banking
Reading Time: 5 minutes
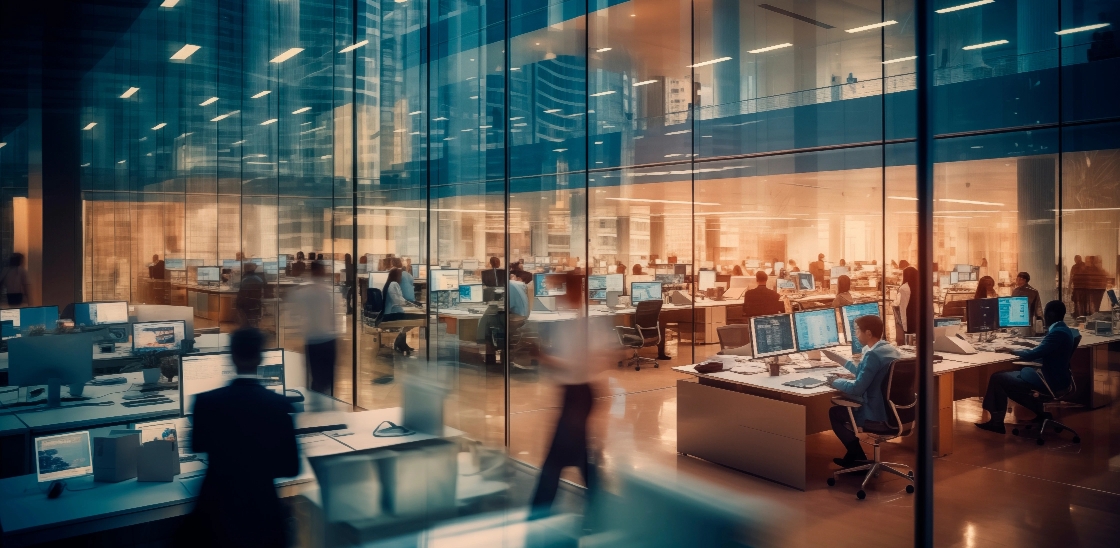
For decades, risk management relied heavily on historical data and reactive measures. Loan approvals were based on heuristic records, fraud detection focused on identifying anomalies after the fact, and market risks were assessed primarily through external reports. This reactive approach, while valuable, has limitations. It leaves blind spots, reacts to problems instead of anticipating them, and struggles to adapt to the ever-evolving financial landscape.
Investment banks must supplement intuition with data-driven systems. It can help them understand key risk factors and impacts in real numbers, and identify future risks long before they materialize. According to a recent Gartner survey, by 2026 organizations that prioritize their security and risk investments based on a continuous threat exposure management program will be 3x less likely to suffer a breach.
Harnessing AI and data analytics for effective risk management
The strategic integration of AI and data analytics into risk management is not merely advantageous but imperative. It empowers banks to swiftly detect, assess, and mitigate risks, ensuring proactive decision-making in the face of uncertainties.
Consider a use case where predictive analytics models, accurately anticipate market fluctuations, enabling timely adjustments to investment portfolios. This not only safeguards financial assets but also positions your organization as a proactive leader in risk management.
90% of banking and financial services companies want to integrate AI tools within compliance and risk management functions.
Source: Moody’s
By consolidating risk data enterprise-wide, banks gain accessibility, visibility, and analytical power to quantify exposures and predict scenarios before they escalate into catastrophic losses.
Understanding data challenges for AI-powered risk management
Many banks struggle with legacy data infrastructures that pose integration or processing challenges. It is essential to pinpoint and address these data challenges directly to ensure stability and resilience.
- Real-time data integration: With fluctuating economic conditions and constantly changing customer behavior, banks need real-time data integration from various sources to make informed risk decisions. It can be achieved using event-driven architectures, data streaming platforms, and APIs. This ensures that risk models and analyses are based on the most up-to-date information.
- Data quality: Inaccurate or inconsistent data across systems, outdated information, and errors in data integration pose significant challenges for reliable risk management. Banks must implement data quality management processes such as data validation, cleansing, and enrichment. Regular audits and validation checks help maintain the accuracy and completeness of the data.
- Data security and governance: Banks handle sensitive customer information which makes data security a top priority. A lapse in regulatory compliance can lead to legal penalties and a loss of customer trust. It is imperative to deploy robust data governance frameworks that are optimized with encryption, access controls, and monitoring systems.
- Talent and expertise: The shortage of skilled professionals with expertise in data analytics, management, risk modeling, and data management poses a significant challenge for banks. A recent study on the state of AI in financial services states that 36% of businesses find it difficult to recruit AI experts such as data scientists. Banks must invest in training programs for existing staff and develop cross-functional expertise that brings together professionals from finance, IT, and data analytics.
Data infrastructure framework for effective risk mangement analytics
This section explores the essential components and structural elements that form the backbone of an effective risk management analytics system. From data collection to analysis and reporting, this framework outlines a structured approach to harnessing the power of data for informed decision-making.
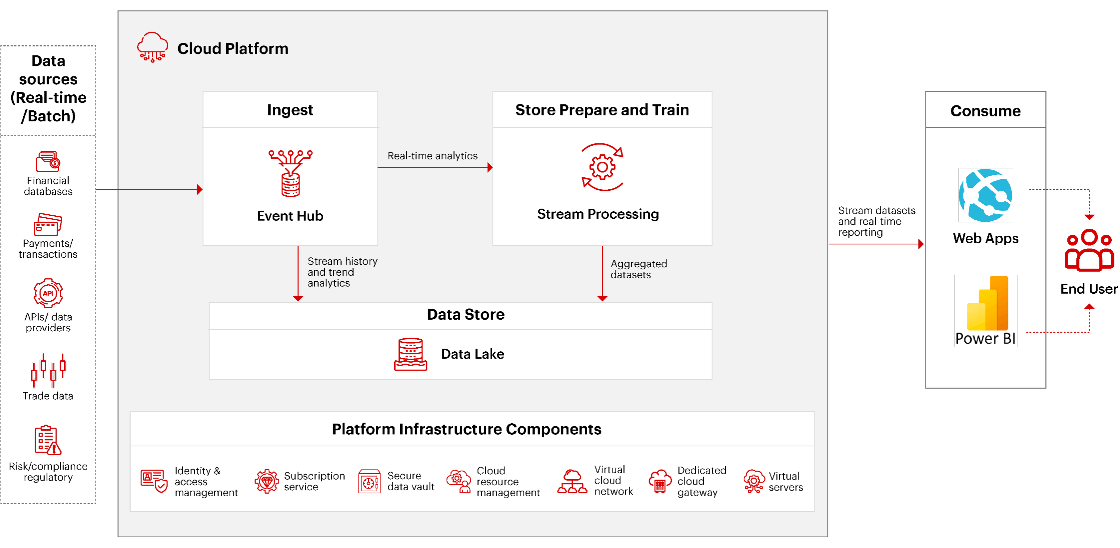
Adapted from Microsoft Azure
Data-driven paradigms shaping risk management in investment bankings
- Cloud-powered advancements: Banking and financial services firms are recognizing the transformative advantages of migrating data to a cloud data warehouse. The transition to cloud-based data architectures empowers them with enhanced scalability and agility to efficiently handle the dynamic nature of risk data. The seamless integration of advanced analytics applications bolsters risk management capabilities through informed decision-making.
- Surge in security measures: With the ever-growing transparency and sharing of data, banks are proactively enhancing their data security frameworks. We can already witness the heightened adoption of data security solutions such as encryption protocols, multi-factor authentication systems, and intrusion detection mechanisms. By combining these data security measures with analytics capabilities, banks can not only safeguard sensitive information but also gain actionable insights.
- Adoption of data driven workflows: Product and services innovations such as digital banking experiences, tailored products, and fintech solutions have broadened the scope of risk management in banks. This is steering banks towards the adoption of data-driven workflows, making processes more agile and precise. These workflows enable a more granular analysis and transform how risks are identified, assessed, and mitigated.
Case in point
Explore how Sigmoid helped a top 3 global investment bank with sophisticated data solutions in mitigating regulatory risk.
Situation | Solution | Impact |
---|---|---|
A leading global investment bank wanted to automate the manual risk-scoring process of its clients and identify the high, medium, and low risks to comply with financial crime compliance (FCC) regulatory requirements. | We built a framework to automate the risk score calculation process. It also helped improve data quality while establishing batch processing and making the system fault-tolerant. |
|
Conclusion
Investment banking demands a shift from traditional, instinct-based risk management to a proactive and data-driven approach. By leveraging predictive analytics and machine learning, banks can anticipate market fluctuations, ensure timely adjustments, and position themselves as leaders in risk management. The current trends and transformations in risk management underscore the need for a unified data management approach and the operationalization of AI/ML tools.
About the Author
Gunasekaran S is the Director of Data Engineering at Sigmoid with over 20 years of experience in consulting, system integration, and big data technologies. As an advisor to customers on data strategy, he helps in the design and implementation of modern data platforms for enterprises in the Retail, CPG, BFSI, and Travel domains to drive them toward becoming data-centric organizations.
Featured blogs
Subscribe to get latest insights
Talk to our experts
Get the best ROI with Sigmoid’s services in data engineering and AI
Featured blogs
Talk to our experts
Get the best ROI with Sigmoid’s services in data engineering and AI