MLOps strategies for scaling enterprise AI initiatives
1. Introduction
A Gartner research shows only 53% of projects make it from artificial intelligence (AI) prototypes to production. This is attributed to the impediments that technology and business leaders face in moving ML models to production. The eBook discusses how to effectively approach MLOps using tried and tested methods.
2. Implications of large-scale ML models in production
The ability to utilize insights from data has extended beyond the realm of influencing business strategy to make operational decisions on a daily basis. The need for advanced analytics systems that aid clear and timely decisionmaking is no longer a ‘good-to-have’ but is a key mandate for justifying business spending.
The appetite to uncover business intelligence from large volumes of data is ever-growing and this has created the need for Machine learning systems to be flexible in accommodating changing data types, scale with increasing data volume, and consistently deliver accurate results despite uncertainties that accompany live data. A recent talk by Mike Gualtieri, Principal Analyst at Forrester, reveals that only 6% of the businesses interviewed have a mature capability to deploy ML models. This primarily refers to the incapability of their ML solutions to be used in production environments with rapidly scaling data.
2.1. Scaling ML models
Since the inception of the Apache Spark Project, MLib has revolutionized the way scalable ML models are created. A major advantage is that it allows data scientists to focus more on scientific research rather than solving complexities (like those of infrastructure and configurations) surrounding distributed data. Data engineers, on the other hand, can focus more on distributed system engineering using Spark’s easy-to-use API and at the same time allowing the data scientists to leverage the scale and speed of the Spark Core. An important note is, that Spark MLib is a general-purpose library containing algorithms for most of the common use cases.
Also, there are full-fledged services of Amazon SageMaker, Google Cloud ML, Azure ML, etc. They not only come with the advantageous feature of auto-scaling but also offer algorithm-specific features like auto-tuning of hyper-parameters, monitoring dashboards, easy deployment with rolling updates, etc
2.2. Debunking the myths about productionizing ML models
Typically the concept of productionizing ML models was assumed to include tasks such as hosting a machine learning model in an API or updating ML models and some of the nuances around it. However, a holistic approach for putting ML models in production or MLOps includes the following:
- Creating a scalable environment for deployment
- Capturing and reporting detailed statistics in a transparent manner
- Model serving – providing API and hosting
- Adopting a reliable experimentation framework
Productionizing machine learning models is a complex process and can face several challenges such as model performance requirements, dealing with explainability, model governance to name a few. New models are created almost daily and teams must carefully evaluate the benefits of new models, effort spent on ensuring data quality, and monitor the performance to make productionizing it less challenging.
To summarize productionizing ML models encompasses all activities to realize tangible business gains and automate machine learning systems while minimizing the associated risks. Deploying and productionizing ML models
2.3. MLOPs for seamless model training and deployment
Organizations today are looking at realizing tangible results of their ML projects. However, several machine learning challenges make it evident that propelling an ML venture to production is no mean feat. That is why best practices to eliminate the hassles of developing an ML model and expedite its deployment have been identified and defined. These best practices are referred to as MLOps.
Only 22% of organizations have been successful in deploying an ML model in the last couple of years, despite increasing the investment, which aptly highlights that it’s easier said than done.
MLOps enables rapid, continuous production of ML applications at scale. It addresses the unique ML requirements and defines a new life cycle parallel to SDLC and CI/CD protocols. This makes an ML model more effective and its workflow more efficient. It comprises the following components that ensure maximum model performance and ROI:
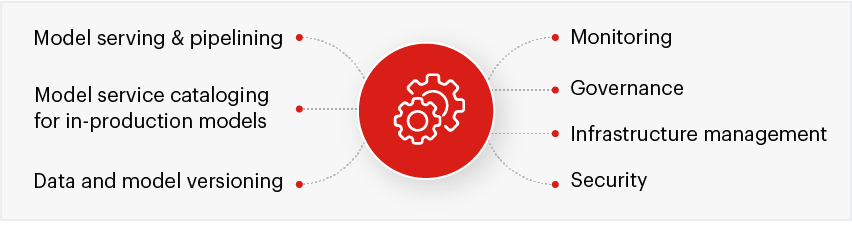
3. Building machine learning models
Creating successful machine learning models consists of various steps such as gathering and cleaning data, visualizing the data, training the algorithm, deploying it and maintaining them. Once all the necessary data is available, models are ready to be built and deployed. We have covered building ML models under three heads in this section — model selection, model testing, and model deployment — in a phase-wise approach.
Model selection
Model selection is the process of selecting one final machine learning model from a collection of candidate ML models for training datasets. Model selection may depend on several factors such as model performance, complexity, maintainability, and available resources or other specific requirements from stakeholders. After a model is selected, model weights are generated and assigned. It helps in mitigating modeling risk, which primarily deals with questions such as:
- Is the data predictive enough to actually do the predictions at this point?
- Does it have enough information to bring about the targeted business change?
Note: Brainstorming with data science teams and being available to them ensures that they select and work with the right models that can be taken to production.
Choosing the right tech stack:
Technologies when selected diligently allow for interoperability across different modeling technologies, if the models are compatible across multiple stacks. Data scientists need to be given the freedom to choose from a range of technology stacks or a range of modeling technologies so that they can explore. At the same time, there should be a keen eye to refrain from technology that makes productionizing the model difficult.
Success criteria
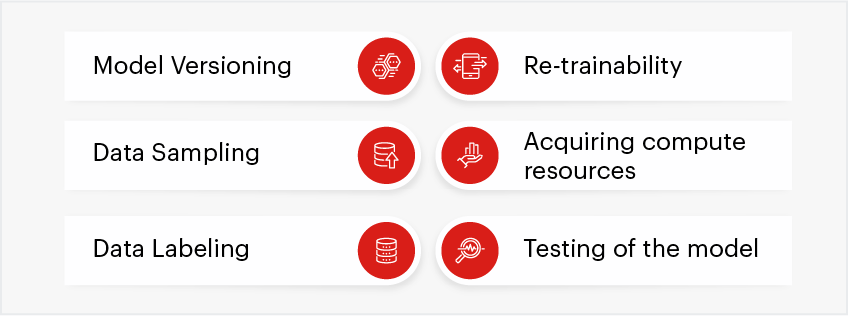
Model training and testing
As models are being built, testing is a critical process. Without rigorous training and validation, an ML model is as good as an engine without fuel. However, training an ML model is tricky, to say the least. This is because it depends on the quality and richness of training and validation data. Replicating the real-life data supply for model training and validation is understandably very difficult. Below are some of the most common challenges in training and validation of an ML model:
- Not enough training data
- Poor quality data
- Overfitting
- Underfitting
- Unrelated Features
Model deployment
Finally, after ensuring that the modeling risks have been eliminated, data is predictive in nature, and the right modeling techniques have been selected which can further be taken into production, the model is ready for the next step – Deployment. Deployment is a very engineering-driven activity. Below are some of the key aspects that need attention to ensure a smooth process.
Codebase: The code base that has so far been written needs to be polished so that it can be battle-tested and put into production.
Integration: Next the proper integration approach needs to be determined. Some questions that need to be answered are:
- Will there be an API endpoint that people are going to fire on and get results from the model?
- Is it going to be a bulk process model that is going to be integrated with ETL tools?
- How to actually orchestrate the workflow? Will there be a cloud scheduler in Google? if Airflow is used, how will it be automated so that it is comfortable for both teams to carry out new integrations into the workflow system?
- How to get detailed access and monitoring, to be able to track SLA reliability
Coding practice:
- What coding practices need to be employed?
Data scientists’ roles:
- Are they going to be involved in the dev stack?
- Will they have full control over the system (something that we typically prefer) or will they just be able to check in code and be able to see production results that are coming out of it?
- How to ensure that data scientists are deeply involved, and it’s not a siloed activity where data scientists hand over to data engineering teams?
Going beyond deployment
Once the models are deployed, it is important to assess how they will be run and monitored in detail.
If there is a sophisticated experimentation system, then it is essential to measure the results of those experiments and update the business teams on the different models that are running along with the results they generate. And it’s very important at this stage to start involving more and more stakeholders to make sure that the results of those models and the ROI of the system are transparently reported for continued operations.
Success criteria
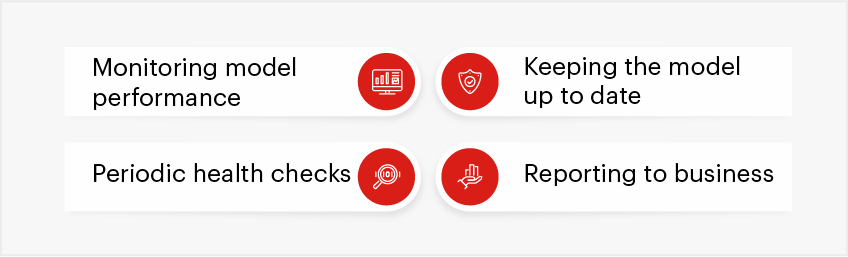
4. MLOPs challenges and ways to overcome them
4.1 Challenges
Despite advancements in tools and technologies, ML modeling is hard to translate into active business gains. There is a plethora of engineering, data, and business concerns that may hinder putting ML models into production. As per a poll conducted by Sigmoid, 43% of respondents said they get roadblocked in ML model production and integration.
Only 53% of projects make it from artificial intelligence (AI) prototypes to production. AI leaders find it hard to scale AI projects because they lack the tools to create and manage a production-grade AI pipeline.
Source: Gartner
The main set of challenges that are covered in this book is those that pose business risks and implementation risks, that Sigmoid has typically addressed while putting ML models into production. The following sections discuss some of the challenges that enterprises face:
Data complexities
ML model training requires about a million relevant records, and it cannot be just any data. Getting relevant data sets fast enough to do accurate predictions isn’t straightforward. Getting contextual data is also a problem. Most ML teams still train ML models on top of their traditional data warehouses, resulting in 80% of the time spent on cleaning and managing data rather than training models. These complexities result in high maintenance costs of running ML models.
Engineering and deployment
Even if the data is available, ML systems can be difficult to engineer. It is also important to standardize different technology stacks so it doesn’t hinder productionizing ML models which is crucial for the model’s success. For instance, data scientists may use tools like Pandas and code in Python. But these don’t necessarily translate well to a production environment where Spark or Pyspark is more desirable. Improperly engineered technical solutions can cost quite a bit.
Model drift
Model drift refers to the degradation of the ML model’s predictive ability which can be caused by changes in the digital environment or changes in variables such as concept and data. Model drift is a common occurrence in machine learning models that happen with time simply by the nature of the machine language model as a whole. Model drift can be of two main types — concept drift and data drift — based on changes in either the variables or the predictors.
Integration risks
A scalable production environment that is well integrated with different datasets and modeling technologies is crucial for the ML model to be successful. Complicated codebases have to be made into well-structured systems ready to be pushed into production. In the absence of a standardized process to take a model to production, the team can get stuck at any stage.
Complex testing requirements
Testing machine learning models is difficult but is an important step of the production process. Running health checks and watching out for data anomalies keep a check on the overall performance of the ML models. However, testing ML models are more complex than software testing. ML-specific testing also includes data and model validation, and trained model quality evaluation.
Lack of skilled resources to drive MLOps
Driving MLOps initiatives requires specifically-skilled resources, such as data engineers and data scientists to perform a number of complex functions such as model development, data assessment, and analysis, and explore ML use cases. There should also be transparent communication across data science, data engineering, DevOps, and other relevant teams to drive ML success. But assigning roles, giving detailed access, and monitoring every team is complex.
Continuous pipeline visibility
ML model deployment requires multi-step pipelines that are critical for automated retraining and deployment. This step is complex as it needs various manual steps in the process to be automated before data scientists and engineers deploy the ML models. This leads to further productionizing challenges due to poor coding and evolving data profiles. It demands continuous tracking of performances to evaluate deviations from expectations to be able to improve the performances.
4.2 Best practices to address MLOps challenges
Data assessment
Data assessment and data feasibility check ensure that data teams have the right data sets to run machine learning models and that they are getting data fast enough to do predictions. Some common issues with enterprise data that should be ironed out before starting to build a machine learning model:
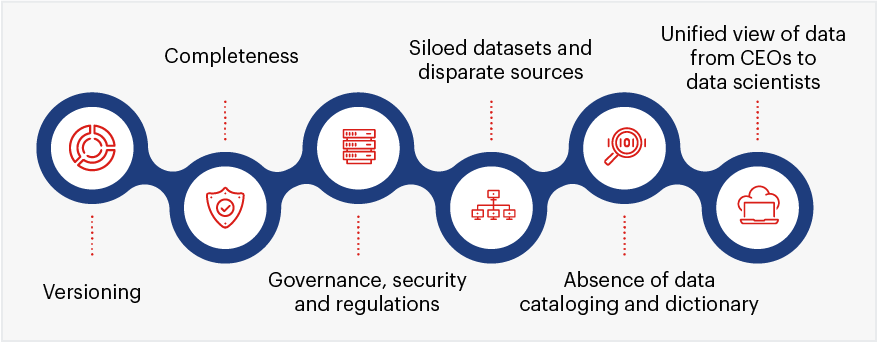
Setting up the right data lake
Building machine learning models on top of traditional data warehouses affects data scientists’ productivity. A data lake environment provides easy and powerful access to a variety of data sources while saving the team a lot of bureaucratic and manual overhead. It provides an opportunity for data scientists to experiment with different data sets and understand what information lies in them in order to be successful.
Evaluation of the right technology stack and scalable compute resources
Selecting the right technology to build and productionize ML models is a crucial step. The data team can pick from a range of technology stacks to experiment with and pick the ones that make ML productionizing easier. The technology chosen should be benchmarked against stability, the business use case, future scenarios, and cloud readiness. Moreover, a scalable computing environment, where they can scale up and down to ETL and process the data analyzed, helps progress much faster and be more productive.
Post-deployment support and testing
Once the ML models are deployed, the environment should be tested in real-time and monitored closely. In a sophisticated experimentation system, test results can be sent back to the data engineering teams to update the models. For instance, the data engineers can decide to overweight the variants that overperform in the next iteration while underweighting the underperforming variants. Negative or wildly wrong results should also be watched out for. The right SLAs need to be met.
Team collaboration and communication
Running successful ML models requires clear communication between the various cross-functional teams to mitigate risks at the right step. While data scientists have to take full control of the system to check in codes and see production results, the DevOps team contributes to maintaining the pipelines. Transparent communication and a strong collaboration between the teams can set up the project for success right from the start.
Role | Responsibilities |
---|---|
Data engineer |
Makes the appropriate data available for data scientists; focuses on data integration, modeling, optimization quality & self-service. They are aware of the technology stacks, advantages, and limitations |
Data scientist |
Identifies use cases, determines appropriate datasets and algorithms, experiment, and builds AI models. Involving Data Scientists early in the process avoids redundancies and enables the creation of large epics, right in the beginning. This allows execution teams to have a clear understanding of completion milestones of each stage of the model building before moving to production |
AI architect |
Is the glue between data scientists, data engineers, developers, operations (DataOps, DevOps, MLOPS), and business unit leaders to govern and scale AI initiatives |
ML engineer |
Deploys AI models through effective scaling and ensuring production readiness, ensures continuous feedback loop |
DataOps engineer |
Is involved in development and deployment to deliver analytics to end-users. Manages tools and processes to support the data infrastructure and has a fair understanding of how models are going into production |
Adapted from Gartner
Building data governance and cataloging system
A strong cataloging system can fully leverage the work that the data science teams do. After the data is cleaned up and structured in the right way combining different data sources together, cataloging, and capturing it correctly allows other data science teams and internal systems that need this useful data. A strong governance system makes it comfortable to share data and allows data science teams to quickly access it. There are a variety of technology systems to help both of these but what separates successful teams from others is the ability to mitigate feasibility risks.
Feasibility risk primarily deals with questions such as:
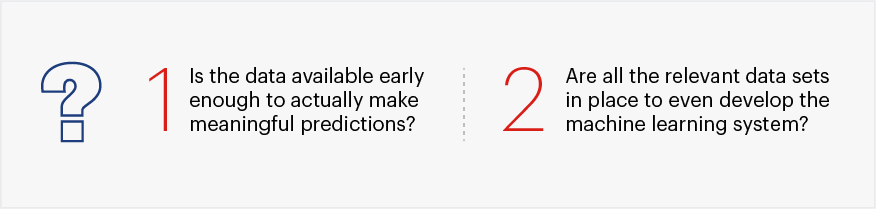
Addressing model drift
Early detection of model drift is critical when it comes to maintaining model accuracy. This is because the model accuracy decreases as time passes and the predicted values continue to deviate further from the actual ones. The further this process goes, the more irreplaceable damage is done to the model as a whole. Hence, catching the problem early on is essential. Refitting models based on past experiences can help to create a predictive timeline for when drift might occur in a model. With this in mind, the models can be redeveloped at regular intervals to deal with an impending model drift.
Have a look at the key technologies & and best practices to productionize ML models at scale
5. Automating MLOps
MLOps practices drive data-driven organizations towards a strategic understanding and adoption of AI and ML. To drive successful MLOPs automation enterprises should take a stock of organizational infrastructures, objectives, and pain points. Below are the steps that organizations can follow to drive successful MLOps automation.
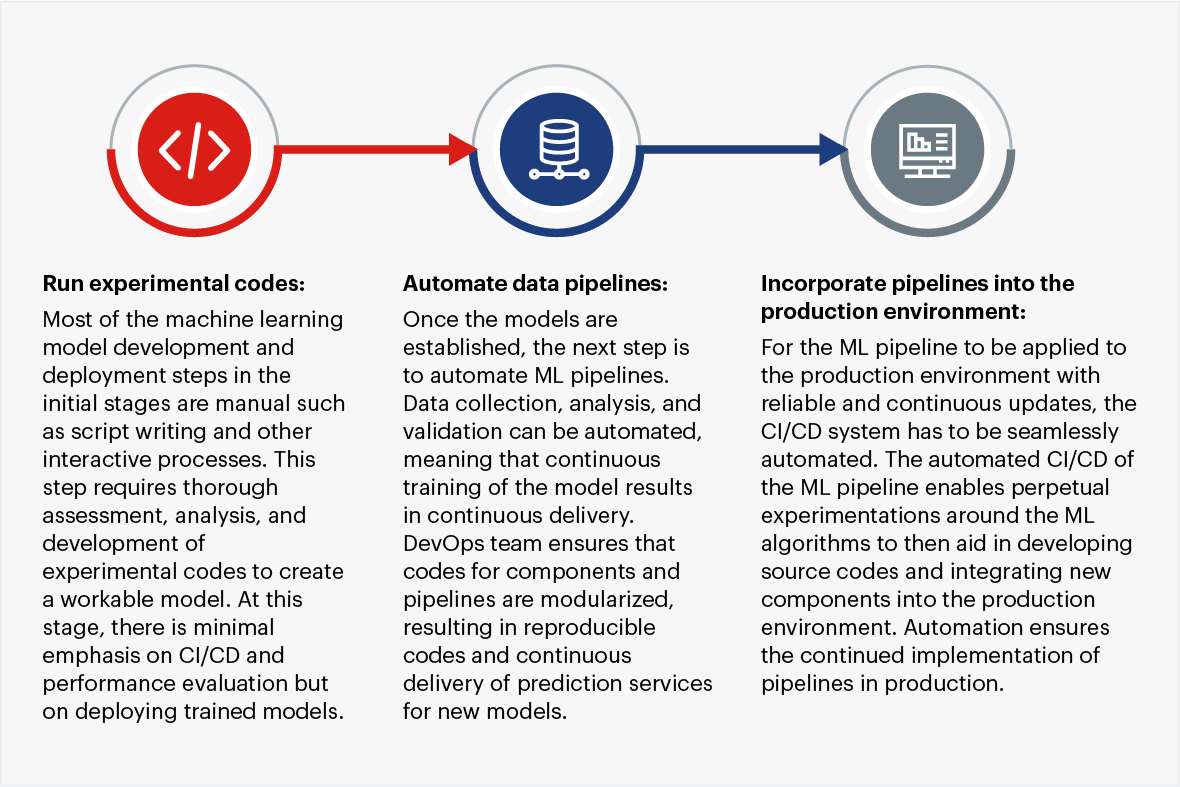
6. Sigmoid’s MLOPs framework
MLOps framework should mindfully incorporate everything from identifying the business requirements to training ML models and maintaining them. Incorporating the right best practices such as those mentioned above, along with leveraging the best talent in data engineering, data science, and DataOps can drive analytics success across the entire ML lifecycle. Sigmoid’s MLOps framework incorporates the practices such as setting up the right data lake and enabling scalable computing resources from the beginning. Monitoring model performance, carrying out periodic health checks and automating model updates to eliminate model drift also help in the long run.
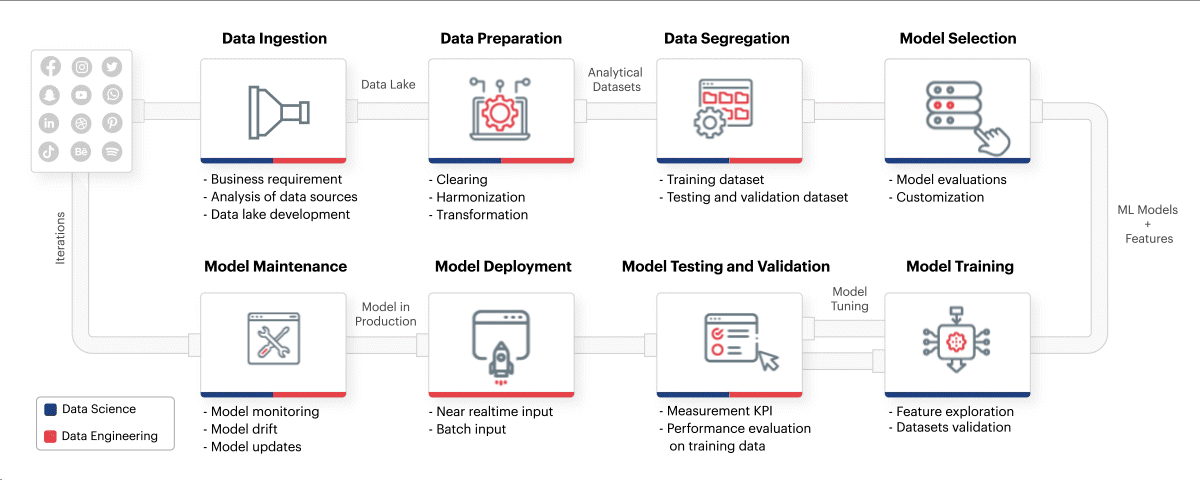
Fig: Sigmoid’s MLOps framework
Seamlessly move your model from concept to production and automate ML pipelines
7. Success stories
8. Key considerations while building scalable ML infrastructure of the future
MLOps has the power to democratize machine learning and empower the community of data practitioners to maximize business impact. It enables ML applications to provide more deeper and consistent insights apart from providing scalability. The idea is to have systems that are sufficiently mature to handle the use case being considered. This ensures that analytics teams focus on their core job and are most productive. Here we discuss a few key considerations that make it up for a good MLOps infrastructure.
Scalable environment for development:
Scaling ML models requires collaborative team efforts to reap unparalleled benefits such as automated and cost-effective ML experiments, better productivity, and reusability. Scalable infrastructure ensures the continuous development of ML models while enabling systems to run even with heavy loads of data.
- Provides access to larger sets of data
- Allows faster turnaround time
- More robust validation before moving to production
- Ensures higher success rates
A fast experimentation framework:
Experimenting focuses on collecting, organizing, and tracking model training information across multiple runs. The fast experimentation cycle allows data teams to try new data processing techniques, model architectures, or parameters and easy benchmarking of the results between experimentations.
- Allows greater agility and experimentation
- Identify errors before they cause issues
- Enable complex but reliable frameworks
- For some use cases like Personalization & Reinforcement, this is mandatory
Model management and serving:
Model management ensures that ML models are consistent and all business requirements are met at scale. It streamlines model training, packaging, validation, deployment, and monitoring to consistently run ML projects.
- Enables self-service for most ML use cases
- Moves the enterprise to a truly data-driven culture rather than a data scientist-driven culture
- Requires disciplined testing
- Addresses common business problems such as regulatory compliance
Detailed and accessible statistics:
Continous monitoring of ML models in production and a summary of statistics of data that built the model, performance of ML models and the insights generated can help the data teams in the long run.
- Better transparency with business
- Better impact analysis
- Less manual effort by data scientists
Conclusion
Data and analytics leaders can greatly reduce the risk of spending time and resources on ML projects that never go into production, by employing a robust framework for MLOps that seamlessly allows the integration of AI solutions with existing live applications. Almost every business relying on data greatly benefits from a scalable technology environment. Most of them need some model serving systems, especially if they are serving out APIs. However, it need not always translate to a very sophisticated investment, especially in the case of bulk processing.
Enterprises need to invest in detailed access to key data, servicing them up to business, and then finally having an experimentation framework. It most certainly depends on the use case and a lot of businesses currently consider this as something that is ‘good to have. But this will soon transform into a ‘must-have’ for any enterprise that is not only looking at uncovering seemingly imperceptible opportunities with data but also enhancing their daily business operations across domains.