Data Pipeline & ETL Data Warehousing Services
Build efficient pipelines and automate data ingestion for faster insightsHome / Data Engineering / Data Pipelines
Integrate data from multiple sources and reduce data latency with ETL for Data warehouse service
To overcome the challenges posed by data silos, Sigmoid’s data pipeline services help to automatically ingest, process, and manage huge volumes of data from diverse sources. We have built over 5000 data pipelines, improved query performance and empowered organizations with faster data access and near real-time visibility to insights. Leveraging our expertise in the end-to-end data engineering ecosystem and open-source technologies, we build flexible ELT solutions by writing cloud-native code. In addition to hand coding data pipelines, Sigmoid builds data pipelines using a combination of no-code, low-code tools and automation.
Building modern data architecture with data lake
Find out how businesses leverage data lakes to capitalize on the available data and drive real-time insights for faster and more effective decision making.
Download guidebook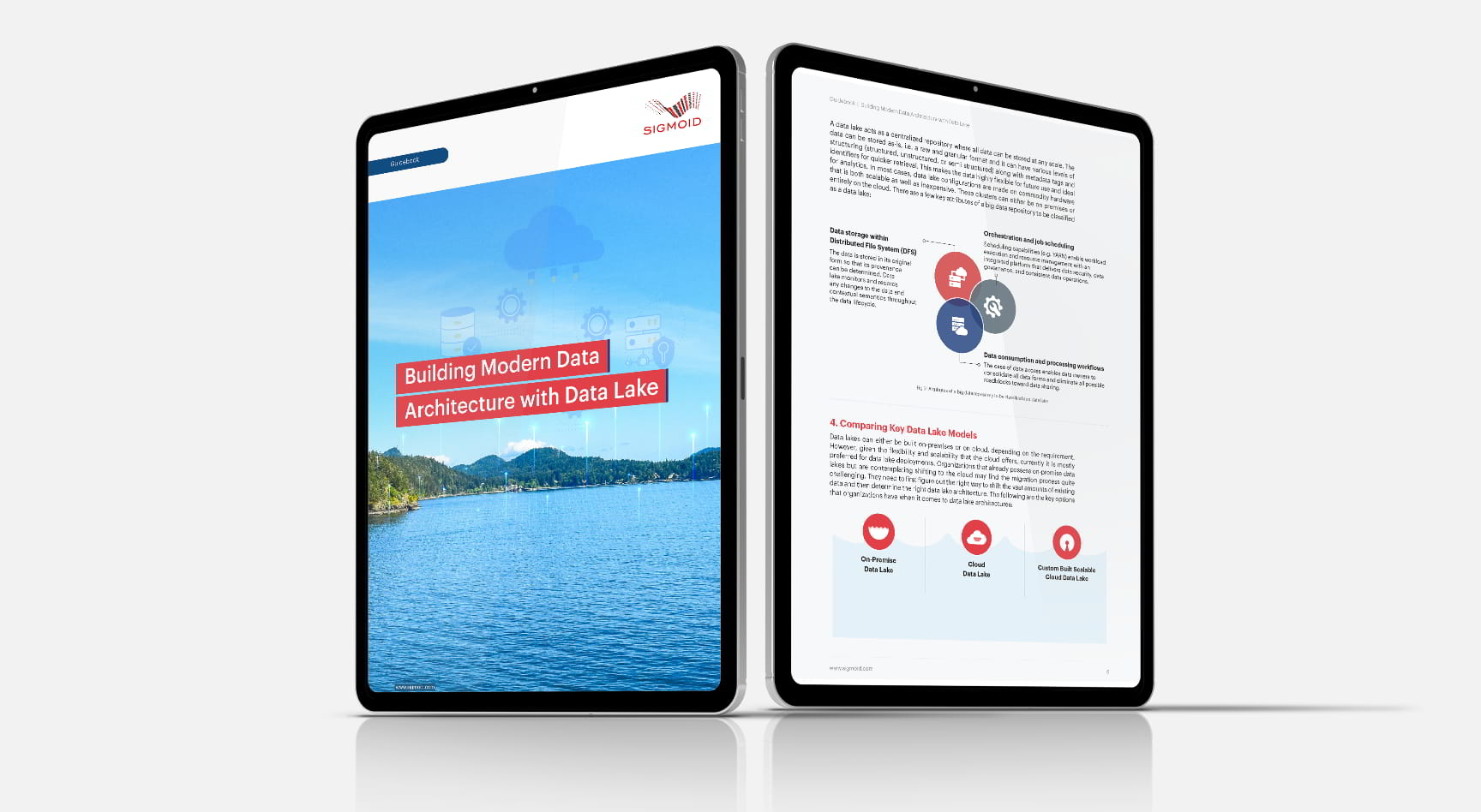
End to End data pipeline development and management services

Ingest
Connect siloed data sources faster with our proven frameworks.

Automate
Automate ingestion and data processing from diverse sources.

Streamline
Efficiently process data for real-time reporting and insights.

Migrate
Migrate to the right cloud infrastructure at optimal cost.

Optimize
Improve query performance and enhance scalability.

Govern
Get robust data lineage, security and compliance.
Get faster access to data with powerful data pipelines tech stack
Our data pipeline management is built upon cloud and open source technologies designed to meet the demands of modern data processing and develop data lakes. This empowers our data engineers to use data warehouses and work seamlessly across various stages of the data lifecycle, from data extraction and integration and ingestion pipelines to transformation and analysis, resulting in a streamlined and faster access to actionable data insights.
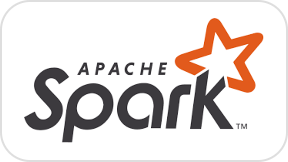
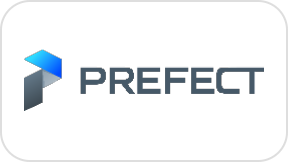
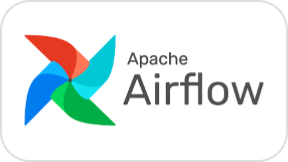
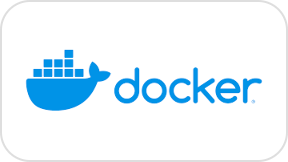
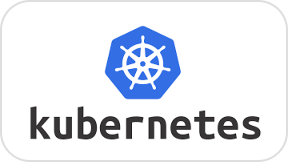
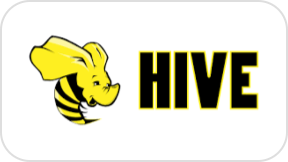
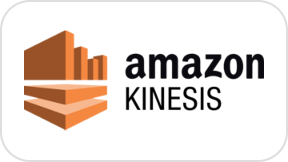
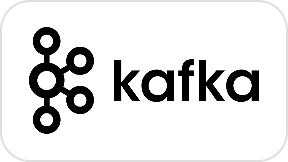
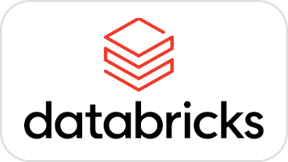
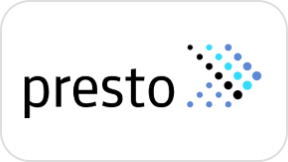
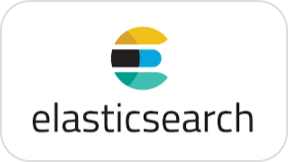
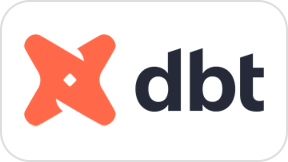
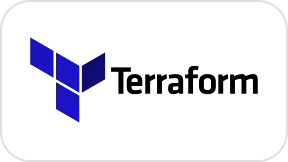
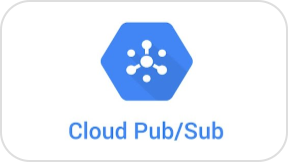
Empower your enterprise to scale and accelerate with data pipeline services
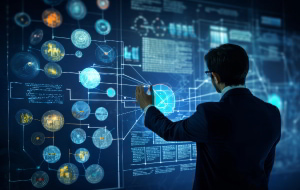
Data ingestion pipelines automate data processing from diverse sources and with our low-code, no-code frameworks. By using cutting-edge technologies, we ensure that data workflows are seamlessly orchestrated to get faster time-to-insights.
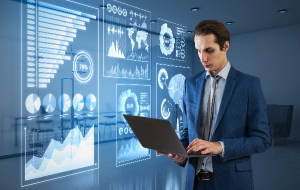
Implement strong data governance measures with Unity Catalog in Databricks, Atlassian and other platforms to ensure robust access control, data lineage tracking, policy enforcement, and other benefits.
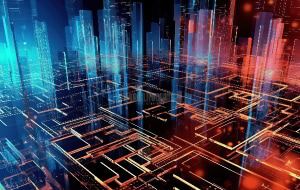
Enable flexible architecture and automatic scaling of resources propagating to multiple environments using Docker and Kubernetes. Seamlessly adapt to dynamic data volumes and processing requirements for optimal performance at all times.
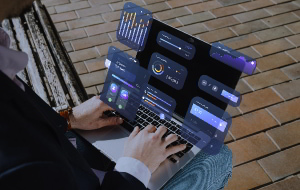
Track the performance, health, and status of data workflows in real-time. Our experts can proactively address any issues using centralized data lake ETL and ensure end-to-end data pipeline visibility.
Customer success stories
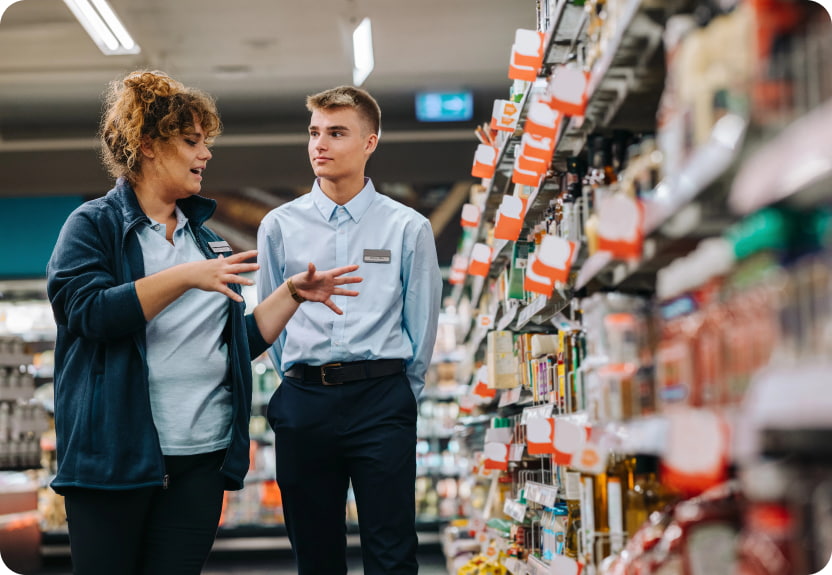
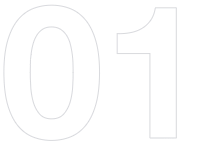
80% performance improvement with scalable data pipelines in an Azure data platform for leading retail data vendor
- 35% YoY cost savings
- 83% reduction in execution time of Spark transformation
- Enhanced reporting and 80% performance improvement
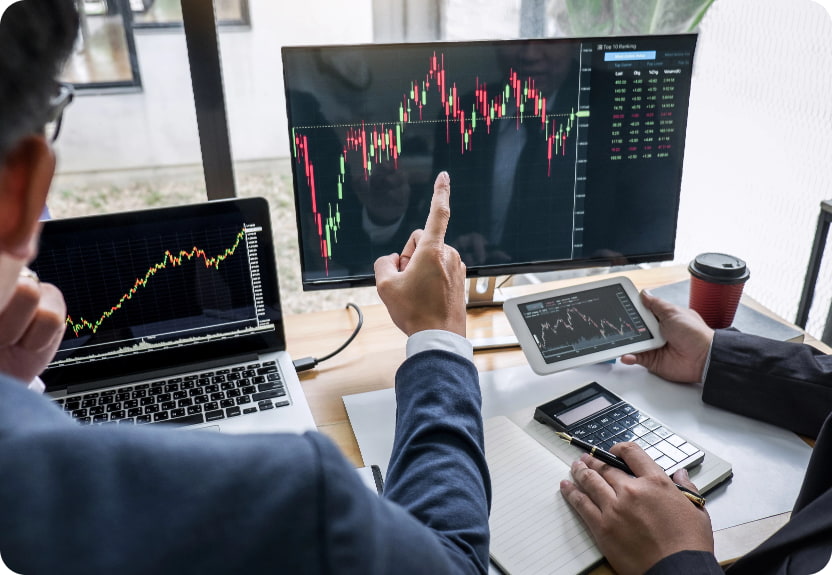
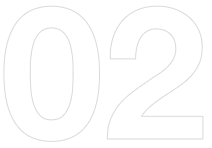
Enhanced trade surveillance and regulatory compliance with 4x faster, efficient data pipelines for a global investment bank
- Processed 100 MN rows of asset class and market data daily
- 65% reduction in false alerts
- Quicker surveillance results
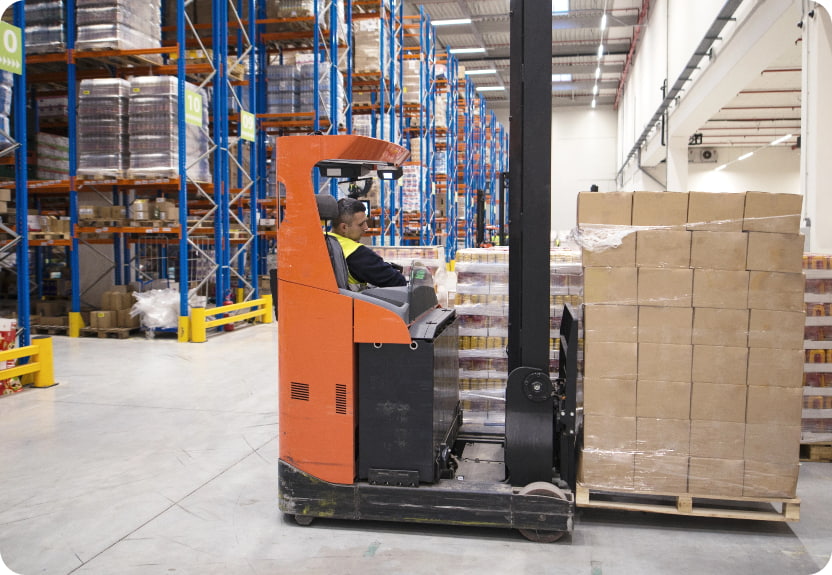
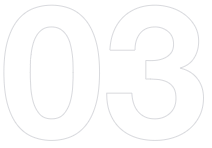
Centralized data lake with automated data ingestion from 30+ sources to enable faster marketing analytics for a major F&B brand
- Automatically ingested data from 30+ sources
- 50% faster data collection and enrichment
- 2.5x faster time to insights for marketing team
Our other offerings in data engineering

ML Engineering
Strengthen ML model lifecycle management and accelerate the time to business value for AI projects with robust ML engineering services.

Cloud Transformation
Modernize, migrate, and optimize cloud data performance with agility and reliability for optimal performance and data quality.

DataOps
Managed services to help you automate end-to-end enterprise data infrastructures for agility, high availability, better monitoring, and support.