MLOps Solutions
Operationalize machine learning lifecycle faster and maximize the impact of advanced analyticsHome / Data Engineering / Ml Engineering
Build, train and deploy ML models at scale with our industrial MLOps solutions and services
Extracting maximum ROI from machine learning models remains a major challenge for companies, as more than 50% of models fail to reach production owing to silos complicating ML model deployment. Sigmoid’s MLOps managed services and solutions combines data science, data engineering, and dataops expertise to build effective AI strategies and deliver business value. Our expertise in open-source and cloud technologies enables you to build custom MLOps solutions and maximize ROI. Our solutions also facilitate the seamless integration of MLOps platforms into existing workflows. We help data-driven companies to accelerate time to business value for AI projects by 30% by strengthening ML model lifecycle management and overcoming model drift challenges, and improving model predictions accuracy.
ML pipelines maintained
ML models in production
Uptime SLA of ML models
MLOps best practices to solve AI/ML production hurdles
Gartner states that on average, only 54% of AI projects make it from pilot to production. This is attributed to the impediments that technology and business leaders face in moving ML models to production. The eBook discusses MLOps best practices to overcome challenges of training, deploying and maintaining model accuracy at scale with a proven framework.
Download MLOps eBook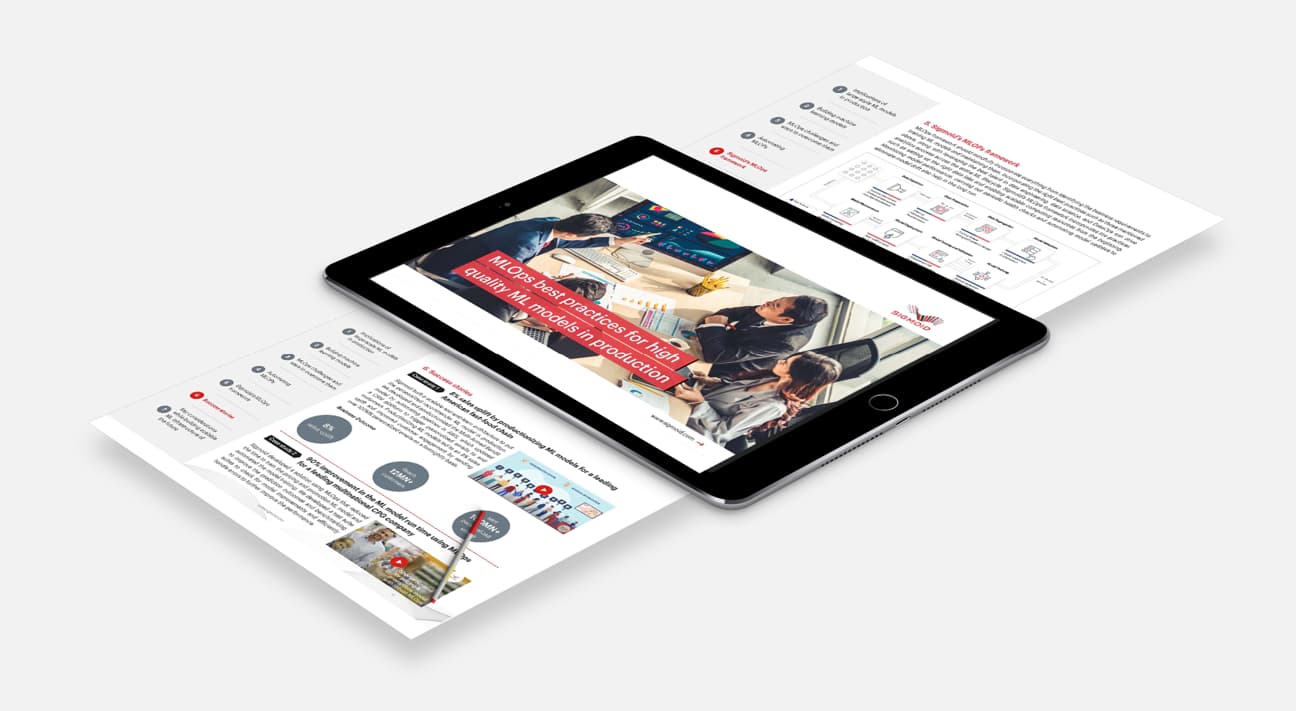
Drive strategic AI initiatives with MLOps Platforms
Our MLOps tech stack is designed to help extract maximum ROI from machine learning, build effective AI strategies, and deliver tangible business value. The end to end MLOps platforms like Azure, Databricks ML, AWS Sagemaker, ML flow, Kubeflow etc. Streamline the entire ML workflow from data preparation and model training to automated model deployment, monitoring and model retraining With our expertise in open-source and cloud technologies we enhance productivity and drive innovation across your organization.
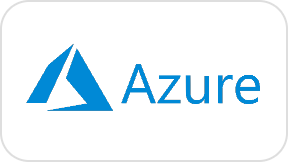
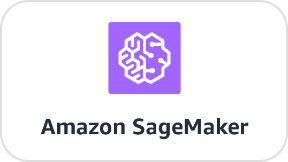
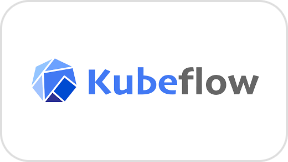
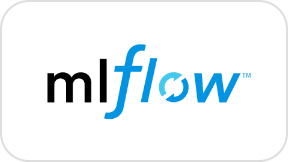
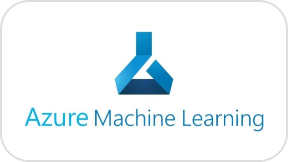
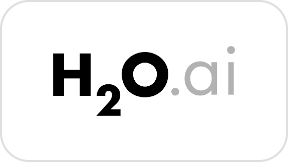
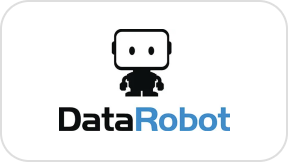
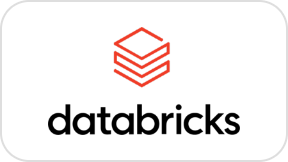
Enhance ML model lifecycle management

Model Building
Facilitate expedited model development through model monitoring, training, and testing, while implementing a model repository and scalable infrastructure provision.

Model Deployment
Maximize AI initiatives and leverage open-source and cloud-based solutions or MLOps tools to deploy scalable MLOps frameworks.

Model Serving
Enable batch or real-time business insights for reports/dashboards and downstream systems, including model fine-tuning, to enhance decision-making.

Model Management
Detect & manage model drift data drift, and model degradation to ensure model accuracy and performance, including monitoring model performance.
Sigmoid’s MLOps framework
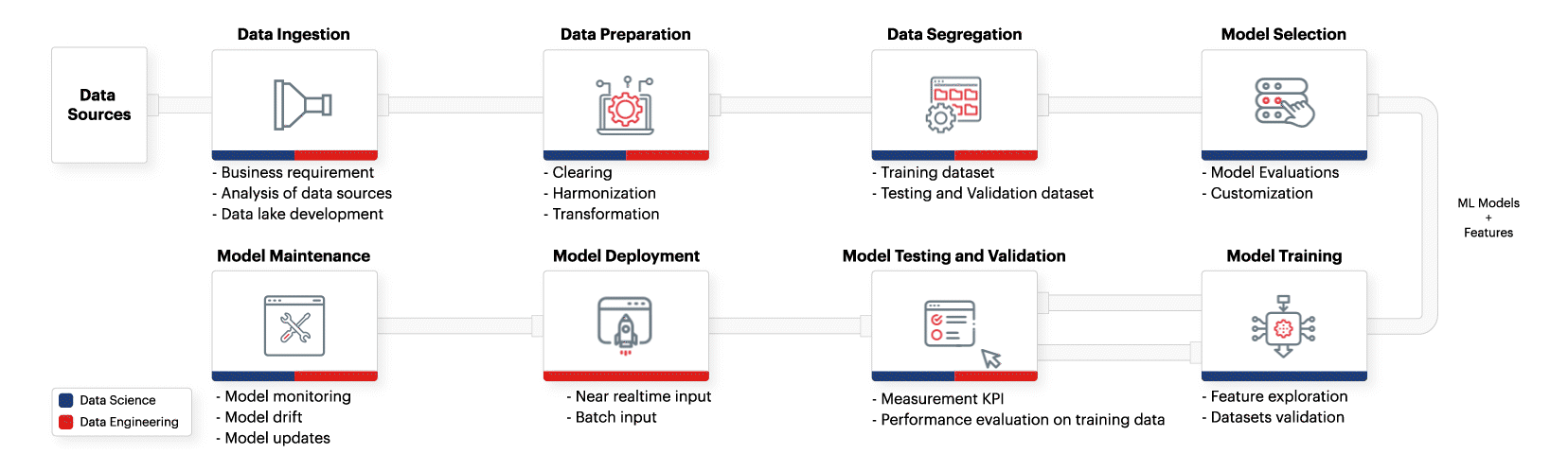
ML customer success stories
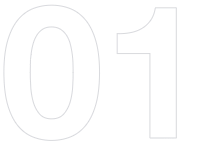
90% improvement in the pricing and promotions ML model runtime for a top hygiene company
- Reduction in model run time from 8 days to just 14 hours
- 87% reduction in cost per run
- Easy and scalable migration of ML models across geographies
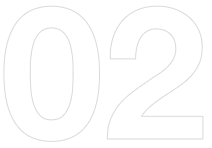
Automated ML pipelines for 1:1 email marketing for a QSR chain
- Over 100 MN personalized emails sent on a fortnightly basis
- 12 MN customer base
- Delivered 8% sales uplift
Our other offerings in Data Engineering

Data Pipelines
Automated data pipeline solutions to generate insights faster and make smarter business decisions.

Cloud Transformation
Modernization, migration, and optimization of cloud performance with agility and reliability for optimal data usage.

DataOps
Managed services to help you automate end-to-end enterprise data infrastructures for agility, high availability, better monitoring, and support.