Data mesh and data fabric: The rise of new data management approaches
Reading Time: 5 minutes
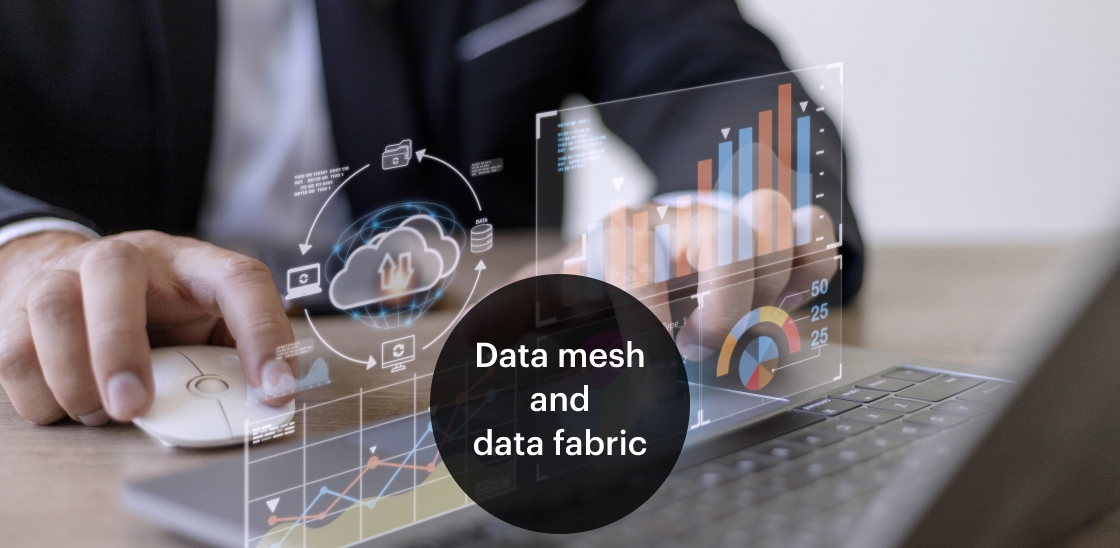
Organizations are increasingly leveraging data-driven insights to elevate their operational efficiency and produce data assets for a competitive advantage. As a consequence, it is imperative to continually refine the overall data strategy and find relevant data management solutions or approaches. Data Mesh and Data Fabric have emerged as two cutting-edge innovations that are helping organizations derive the maximum value from their data. Data and analytics leaders moving towards modern data approaches, need to fully understand these concepts before deciding on the fitment.
First things first: what is data mesh and data fabric?
Data Mesh
Data mesh is a decentralized data architecture that organizes data as a product, owned by a specific business domain. This means that each business domain is responsible for hosting, preparing, and serving data to its own domain along with a broader audience. It fosters flexibility and autonomy among data teams, empowering them to develop, manage and maintain the quality of their data products. As a solution architecture aimed at building business-oriented data products, data mesh greatly emphasizes data ownership and accountability across the organization. One significant advantage of data mesh is its ability to make enterprises more agile.
Data Fabric
Data fabric represents a centralized approach to data management, and can be considered as a cohesive layer of data and connecting processes. This architectural approach advocates for establishing a unified data layer to serve as the single source of truth. Data fabric leads to data quality, consistency, and security by streamlining the process of data access and management between various teams. However, a data fabric largely differs from traditional data warehousing or data lakes. Implementing a data fabric fosters better collaboration between multiple teams and departments, ensuring they can access and utilize the same data consistently.
Weighing the benefits of data mesh and data fabric as modern architecture approaches
Both data fabric and data mesh are revolutionary architectures, addressing the issue of growing data complexities. Data mesh and data fabric are enabling a whole new set of business outcomes from customer experience to productivity and revenue maximization through the following attributes:
Attribute | Data mesh | Data fabric |
---|---|---|
Product orientation Vs. people orientation | Regards data as a product, with consumers accessing this data to create value, aiming to reduce access friction for both technical and non-technical users. | Relies on automation and various tools to connect data across locations and derive insights, focusing on the technical aspects. |
Centralized vs. decentralized culture/structure | Takes a decentralized approach, with multiple teams managing the data they own, ensuring better use of data products across multiple domains. It offers greater autonomy for the teams in daily operations, fostering cross-functional collaboration. | Adopts a centralized approach, with a single team or department managing and governing the data infrastructure. It is suitable for a centralized organization with a more hierarchical structure, improving alignment of the teams with the data they use. |
Complex vs. simple data ecosystems | Decentralized access in data mesh helps to navigate complex data ecosystems at scale. | It is better suited for simpler, unified data architectures with a single source of truth. |
Unified vs. modular structure | Modular with independent teams managing their data and making it available through objective-based APIs or Software Development Kits (SDKs). | Unified and integrates data services into a singular environment accessible via controlled datasets. |
Connected vs. distributed architecture | A distributed pattern leading to creation of specialized domains within an organization. | A technology-driven metadata approach using tools and technology stacks to connect data sources for end-user access through self service. |
Organizational vs. team governance | Data governance lies with individual teams, thus promoting decentralized governance. It enhances ownership and accountability at the team level. Higher focus lies on data quality and management. | Empowers the organization to centrally manage and govern data, improving data quality and reducing data silos. It promotes consistent and unified data management practices with uncompromised data security. |
While there are some complexities involved with data mesh and data fabric, they can both be used to greatly enhance end-to-end data management. Based on the attributes of the two approaches and its alignment with the overall business goals, data or analytics leaders can choose the most suitable framework for their enterprise.
Data mesh and data fabric are complementary in nature
A data mesh and a data fabric could exist simultaneously in the same organization- it need not be an either/or proposition. In fact, data fabric can facilitate the implementation of data mesh in several ways and data mesh can further data fabric objectives:
- Data owners are equipped with tools to create data products, including cataloging data assets, transforming into products, and establishing federated governance policies
- Data owners and consumers can utilize data products by publishing them to a catalog, searching for and discovering data products, and querying or visualizing them using data virtualization or APIs
- Insights derived from data fabric metadata can be harnessed to automate tasks by learning from patterns during creation and monitoring data products
Data fabric offers flexibility to start with specific use cases to achieve quick time-to-value, regardless of where the data resides. Organizations can leverage the capabilities offered by data fabric to implement a data mesh across various stages. A data fabric provides the ability to implement and fully utilize a data mesh by automating new data product creation and data lifecycle management. For example, enterprises can bring machine learning applications closer to their end users by automating data preparation stages of the ML process. This enhances model speed and accuracy, and makes the model available for consumption through controlled datasets.
By leveraging the fluidity of a data fabric foundation, enterprises can implement a data mesh while maintaining a use case-centric data architecture.
Future proof your data management with new-age approaches
Getting started on next generation data architecture approaches almost always requires starting with a clear understanding of business- strategic goals, key stakeholders, pain points in the current environment, financial constraints, available resources, and costs of the current ecosystem. Data leaders also need to assess the current data management practices, culture and technology readiness. Once the groundwork is done, we need to find the right tools for quality control and establish a mechanism for continuous improvement. The choice between data mesh and data fabric ultimately depends upon the approach that aligns best with your data goals, resources, and strategic vision.
Featured blogs
Subscribe to get latest insights
Talk to our experts
Get the best ROI with Sigmoid’s services in data engineering and AI
Featured blogs
Talk to our experts
Get the best ROI with Sigmoid’s services in data engineering and AI