Apache Spark for real-time analytics
Reading Time: 4 minutes
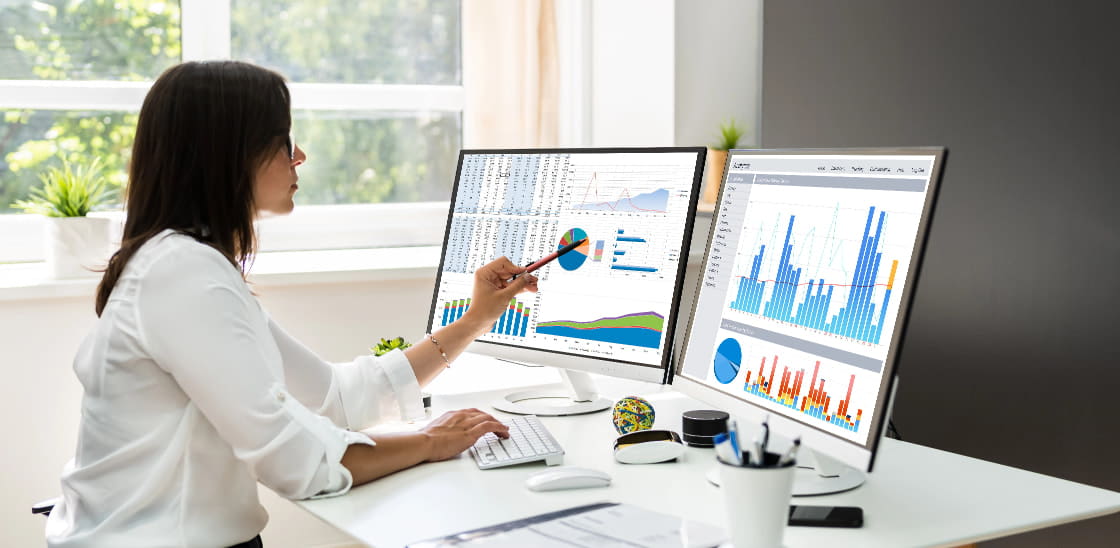
Apache Spark is the hottest analytical engine in the world of Big Data and Data Engineering. Apache Spark architecture is largely used by the big data community to leverage its benefits such as speed, ease of use, unified architecture, and more. Apache Spark has come a long way from its early years to today where researchers are exploring Spark ML. In this article, we will cover Apache Spark and its importance, as part of Real-Time Analytics.
Apache Spark is an open-source fast engine, for large-scale data processing on a distributed computing cluster. It was initially designed at Berkeley University and later donated to the Apache software foundation. Spark can interactively be used from Java, Scala, Python, and R among others, and is also capable of reading from HBase, Hive, Cassandra, and any HDFS data source. Its interoperability and versatile nature make Apache Spark one of the most flexible and powerful data processing tools available today.
Apache Spark architecture is well suited for data cleansing, data wrangling, and ETL. It has an advanced DAG execution engine that supports acyclic data flow and in-memory computing, which helps to run programs up to 100x faster than Hadoop MapReduce in memory. Spark is a multi-stage RAM-capable cluster-computing framework, which can perform both batch processing and stream processing. It has libraries for machine learning, interactive queries, and graph analytics, which can run in Hadoop clusters through YARN, MESOS, and EC2, while it has its own standalone mode. Spark batch processing applications provide high volume as compared to real-time processing, which provides low latency.
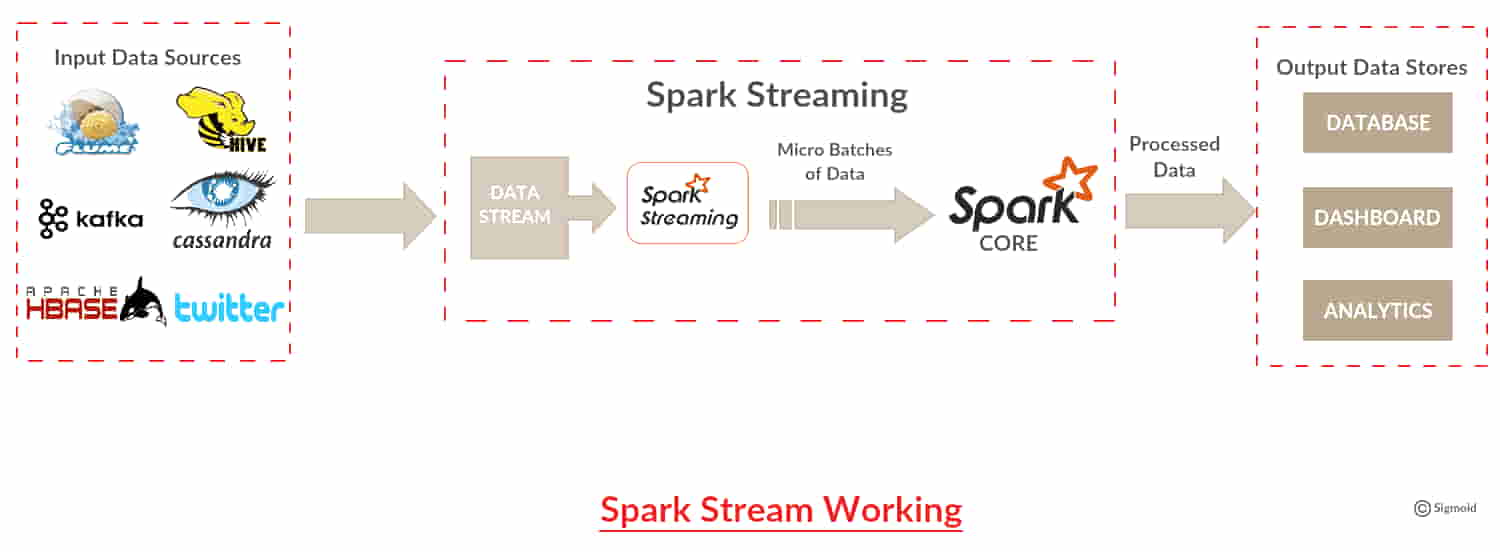
While using Hadoop for data analytics, many organizations figured out the following concerns:
- MapReduce Programming is not a good match for all analytics problems, as it isn’t efficient for iteration and interaction analytics.
- It was getting increasingly difficult to find entry-level programmers with good Java skills, to be productive with MapReduce.
- With the emergence of new tools and technology, fragmented data security issues emerged, which resulted in Kerberos authenticated protocol.
- Hadoop lacked full-feature tools for data management, data cleansing, governance, and metadata.
Apache Spark solves the above concerns:
- Spark uses Hadoop HDFS as it doesn’t have its own distributed file system. Hadoop MapReduce is strictly disk-based, whereas Spark can use memory as well as the disk for processing.
- MapReduce uses persistent storage, whereas Spark uses Resilient Distributed Datasets (RDDs) which can be created in three ways: parallelizing, reading a stable external data source such as HDFS file, and transformations on existing RDDs.
We can process these RDDs using the operations like map, filter, reduceByKey, join and window. The results are stored in the data store for further analytics, which is used for generating reports and dashboards. A transformation will be applied to every element in RDD and RDDs are distributed among the participating machines. Partition in RDD is generally defined by the locality of the stable source and can be controlled by the user through Repartitioning.
Time and speed are of key relevance when it comes to business decisions. To make relevant business decisions, Big Data is ingested in real-time and insightful values must be extracted upon its arrival. So, there are different streaming data processing frameworks like Apache Samza, Storm, Flink, and Spark Streaming.
Here are our top 5 picks of Apache Spark Streaming applications:
- Real-Time Online Recommendation
- Event Processing Solutions
- Fraud Detection
- Live Dashboards
- Log Processing in Live Streams
Wrapping Up
Apache Spark Stream is most suitable for high speed and real-time analytics, which makes it the most sought-after technology in the Big Data world. Complex machine learning algorithms are built and implemented on different streaming data sources through Apache Spark to extract insights and help detect anomalous patterns with real-time monitoring. Through the Spark Streaming library, it is now possible to process and apply complex business logic to these streams.
Apache Spark architecture allows a continuous stream of data by dividing the stream into micro-batches called Discretized stream or Dstream, which is an API. Dstream is a sequence of RDDs that are created from input data or from sources such as Kafka, Flume, or by applying operations on other Dstream. RDDs thus generated can be converted into data frames and queried using Spark SQL. Dstream can be subjected to any application that can query RDD through Spark’s JDBC driver and stored in Spark’s working memory to query it later on-demand of Spark’s API.
So, now we understand how the Spark Streaming library can be used for processing real-time data. This library is important for data processing, which plays a pivotal role in providing real-time insights.
About the Author
Raghavendra is the Assistant Marketing Manager at Sigmoid. He specializes in content marketing domains, digital and social media marketing.
Featured blogs
Subscribe to get latest insights
Talk to our experts
Get the best ROI with Sigmoid’s services in data engineering and AI
Featured blogs
Talk to our experts
Get the best ROI with Sigmoid’s services in data engineering and AI